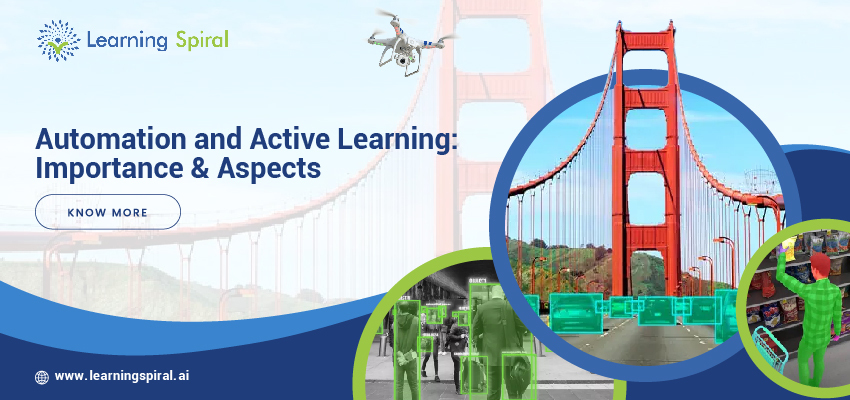
Automation and active learning are two powerful techniques that are increasingly being used together to improve the efficiency and accuracy of machine learning models. Automation refers to the use of technology to perform tasks without human intervention, while active learning is a machine learning technique that involves selecting the most informative data points to label.
By combining these two approaches, it is possible to achieve significant benefits in terms of time, cost, and performance.
The Role of Automation
Automation can be used to streamline various aspects of the machine learning process, including data collection, preprocessing, feature engineering, and model training.
For example, automated data pipelines can be used to collect and clean data from different sources, while automated feature engineering techniques can be used to extract relevant information from raw data.
Additionally, automated hyperparameter tuning can be used to optimize the performance of machine learning models.
The Benefits of Active Learning
Active learning is a valuable technique for improving the efficiency of machine learning models by focusing labeling efforts on the most informative data points.
By carefully selecting which data points to label, active learning algorithms can help to reduce the overall labeling effort required to achieve a desired level of performance. This can be particularly beneficial in situations where labeling data is expensive or time-consuming.
The Synergy Between Automation and Active Learning
The combination of automation and active learning can lead to significant benefits in terms of efficiency, accuracy, and scalability. Automation can be used to streamline the data labeling process, while active learning can be used to focus labeling efforts on the most informative data points.
This can help to reduce the overall cost and time required to train machine learning models, while also improving their performance.
Real-World Applications
The combination of automation and active learning has been successfully applied in a variety of real-world applications, including:
- Medical image analysis:
Automated data pipelines can be used to collect and preprocess medical images, while active learning can be used to focus labeling efforts on the most informative images for diagnosis.
- Natural language processing:
Automated text mining techniques can be used to extract relevant information from text data, while active learning can be used to select the most informative sentences or paragraphs for labeling.
- Autonomous vehicles:
Automated data collection and labeling techniques can be used to train self-driving cars, while active learning can be used to focus labeling efforts on the most challenging driving scenarios.
Future Directions
As machine learning continues to evolve, we can expect to see even more sophisticated applications of automation and active learning. For example, the development of more advanced automation techniques may enable us to automate even more complex tasks, such as feature engineering and model selection.
Similarly, advances in active learning algorithms may allow us to select even more informative data points for labeling, further improving the efficiency and accuracy of machine learning models.
Have any queries regarding the topic? Comment and Leaning Spiral will help resolve your doubts soon.