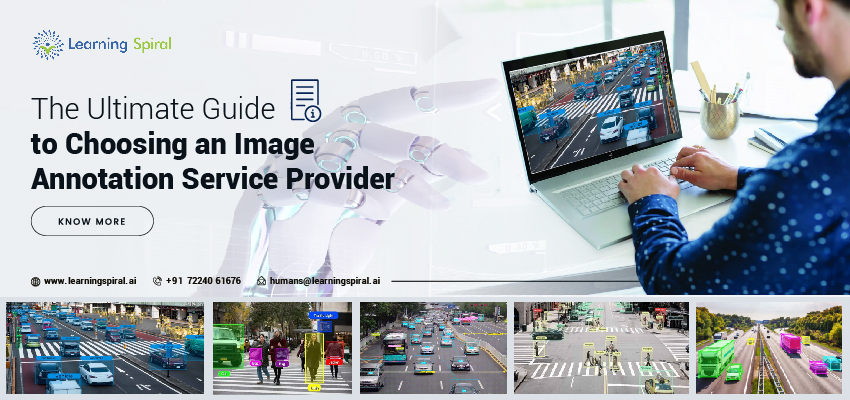
Data is super essential for the advancing world with ever increasing AI presence. But raw data isn’t enough – for AI models to function effectively, they require high-quality labeled data. This is where image annotation services come into play.
These specialized providers meticulously tag images with information, enabling machines to “understand” visual content. Choosing the right image annotation service provider is crucial for the success of your AI project.
Here’s a comprehensive guide to navigate this critical selection process:
1. Understand Your Needs:
The first step is to have a clear understanding of your specific project requirements. This includes:
- Type of Annotation: Do you need bounding boxes for object detection, polygon annotations for intricate shapes, keypoint annotation for pose estimation, or semantic segmentation for pixel-level classification?
- Data Volume: The amount of data requiring annotation significantly impacts project timelines and cost.
- Data Complexity: The complexity of your images (e.g., blurry, low-light) may influence the expertise required from the service provider.
- Desired Accuracy: The level of precision needed for your AI model will influence the annotation process and pricing strategies.
- 2. Evaluate Provider Expertise:
Look for service providers with a proven track record in your specific industry or use case. Consider these factors:
- Project Portfolio: Review their past projects to see if they’ve handled similar data types and complexities.
- Client Testimonials: Seek feedback from previous clients about their experience with the provider’s quality, communication, and efficiency.
- Team Experience: Ensure they have a team of experienced and well-trained annotators who understand the nuances of your project requirements
3. Assess the Quality Assurance Process:
Accurate annotations are paramount for robust AI models. Here’s what to consider:
- Annotation Guidelines: Do they have clear and comprehensive annotation guidelines that align with your project needs?
- Quality Control Measures: Inquire about their multi-stage quality control processes to ensure consistent and accurate annotations.
- Inter-Annotator Agreement (IAA): Evaluate their methods for measuring IAA, which indicates the level of consistency between annotators.
4. Security and Data Privacy:
As data security is paramount, especially for sensitive information, assess the provider’s security measures:
- Data Storage: Ensure they utilize secure cloud storage solutions with robust encryption protocols.
- Data Access Controls: Verify that only authorized personnel have access to your data and that strict access control measures are in place.
- Compliance with Regulations: Confirm that their data handling practices comply with relevant regulations, such as GDPR or HIPAA.
5. Pricing and Service Models:
Image annotation pricing can vary depending on factors like data volume, complexity, and desired turnaround time. Consider these models:
- Per-Image Pricing: Simpler projects might benefit from this model, where each image has a set cost.
- Per-Annotation Pricing: For complex annotations, costs might be based on the number of annotations per image.
- Hybrid Model: A combination of the above, offering flexibility for projects with varying annotation complexities.
6. Communication and Support:
Clear and consistent communication is crucial throughout the annotation process. Look for providers who offer:
- Dedicated Project Manager: A dedicated point of contact ensures smooth communication and addresses any concerns promptly.
- Regular Progress Reports: Transparency is key. Request regular updates on project progress and quality control metrics.
- Flexible Communication Channels: Multiple communication options such as email, project management tools, or even video conferencing facilitate efficient collaboration.
7. Trial Run and Contractual Agreements:
Before committing to a large-scale project, consider a pilot project with a smaller dataset. This allows you to assess the provider’s quality, communication style, and overall fit.
Finally, ensure a clear and concise contract outlines:
- Project Scope: A detailed description of tasks, deliverables, and timelines.
- Pricing Structure: Clearly defined pricing based on the chosen model and any potential additional fees.
- Data Ownership and Security: Explicit agreements regarding data ownership, storage, and access controls.
Conclusion:
Choosing the right image annotation service provider is a critical step in building successful AI models. By considering the factors outlined in this guide, you can ensure you find a partner that offers the expertise, quality, and security needed to fuel your AI initiatives and propel your project towards success.