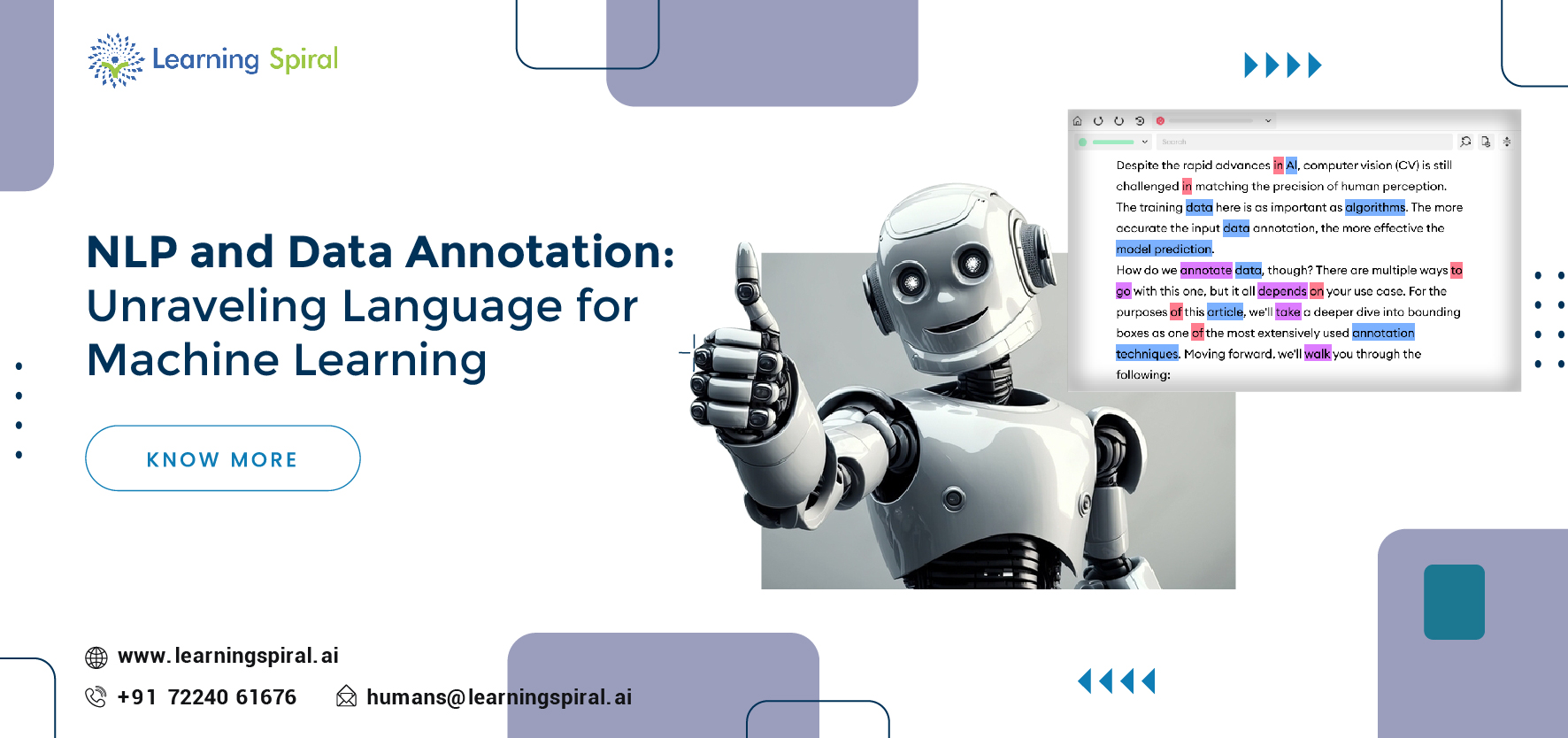
In the realm of machine learning, Natural Language Processing (NLP) and data annotation are integral to creating intelligent systems that understand and interpret human language. As machine learning continues to advance, the need for accurate and comprehensive data annotation becomes increasingly crucial.
NLP focuses on the interaction between computers and human languages. It enables machines to process and analyze large amounts of natural language data, allowing them to understand, interpret, and generate human language in a meaningful way. However, for NLP models to achieve high accuracy, they require meticulously annotated data. This is where data annotation comes into play.
Data annotation involves labeling and tagging data to provide context that machines need to learn and make predictions. In NLP, this can include tasks such as sentiment analysis, entity recognition, and text classification. By annotating text with labels that indicate parts of speech, named entities, or sentiment, we provide the model with the information necessary to understand and respond appropriately to new inputs.
For instance, consider sentiment analysis, where the goal is to determine the emotional tone behind a piece of text. Annotators label texts as positive, negative, or neutral, helping the model learn the nuances of language and context. Similarly, in named entity recognition, entities such as names of people, places, or organizations are tagged, allowing the model to identify and categorize these entities in new texts.
At Learning Spiral AI, our expertise in data annotation ensures that your NLP models are trained on high-quality, accurately labeled data. This precision enhances the performance of machine learning algorithms, leading to more effective and reliable NLP applications. Whether you’re developing chatbots, virtual assistants, or sentiment analysis tools, our data annotation services provide the foundation needed to unlock the full potential of NLP.
In summary, NLP and data annotation work hand in hand to enable machines to understand and process human language. By investing in high-quality data annotation, you ensure that your NLP models are well-equipped to handle complex language tasks, paving the way for advanced and intelligent language technologies.