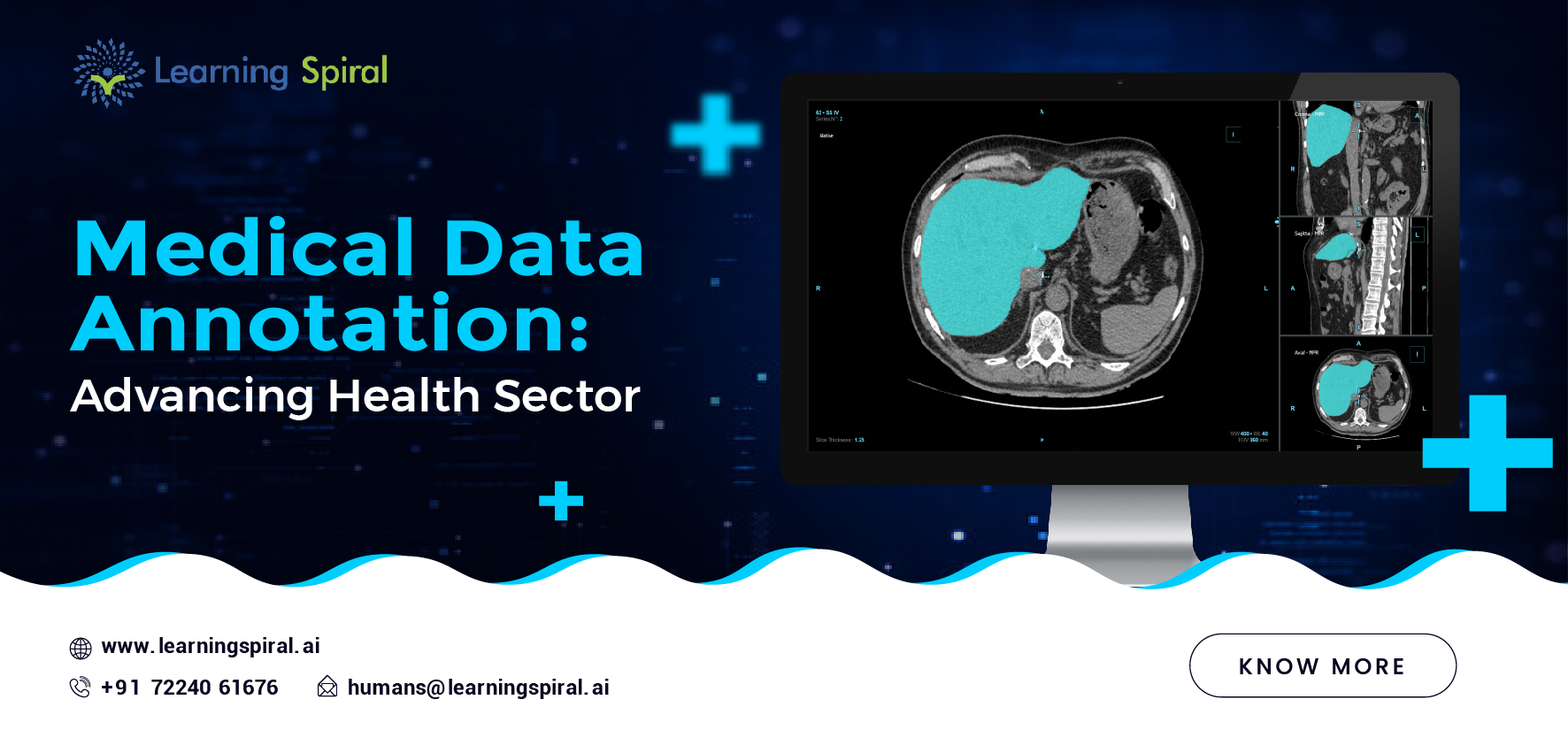
Image annotation, the process of labeling medical images with specific information, is a critical step in developing AI-driven solutions for healthcare.
This meticulous task involves adding metadata to images, such as X-rays, CT scans, MRIs, and ultrasounds, to make them machine-readable and trainable.
The Importance of Precision
Medical image annotation demands an unparalleled level of accuracy and precision. Unlike other domains, errors in medical image annotation can have severe consequences. For instance, incorrectly labeling a tumor as benign could lead to delayed or incorrect treatment. Therefore, it’s imperative that medical image annotation is performed by highly skilled professionals with expertise in the medical field.
Types of Medical Image Annotation
Several types of image annotation are commonly used in the medical domain:
- Image Classification: Assigning a label to an entire image, such as “normal” or “abnormal.”
- Object Detection: Identifying and labeling specific objects within an image, like tumors, organs, or anatomical structures.
- Image Segmentation: Pixel-level labeling to delineate specific regions of interest, such as tumor boundaries or tissue types.
- Landmark Annotation: Identifying and marking specific points on an image, such as anatomical landmarks or tumor markers.
Applications of Medical Image Annotation
The applications of medical image annotation are vast and far-reaching:
- Disease Detection: Training AI models to accurately detect diseases like cancer, heart disease, and neurological disorders.
- Drug Discovery: Identifying potential drug targets and optimizing drug development processes.
- Surgical Planning: Creating detailed 3D models of patient anatomy for surgical planning and simulation.
- Medical Image Analysis: Analyzing medical images to extract quantitative data for research and clinical decision-making.
Challenges and Considerations
While medical image annotation holds immense potential, it also presents challenges:
- Data Privacy: Protecting patient privacy is paramount. Strict adherence to data protection regulations is essential.
- Expert Annotators: Accurate annotation requires medical professionals with deep domain expertise.
- Data Volume: The vast amount of medical image data can be overwhelming. Efficient annotation processes are crucial.
- Annotation Consistency: Maintaining consistency in annotation guidelines is vital for training reliable AI models.
Conclusion
Image annotation is an indispensable component of the medical AI ecosystem. By providing accurate and detailed labels for medical images, it empowers AI algorithms to revolutionize healthcare. As technology advances, we can expect even more sophisticated image annotation techniques and broader applications in the medical field.
By addressing the challenges and adhering to ethical guidelines, the medical community can harness the full potential of image annotation to improve patient care, accelerate research, and ultimately save lives.
Learning Spiral AI has a dedicated team for medical data annotation projects, with over 5 years of experience handling such projects.