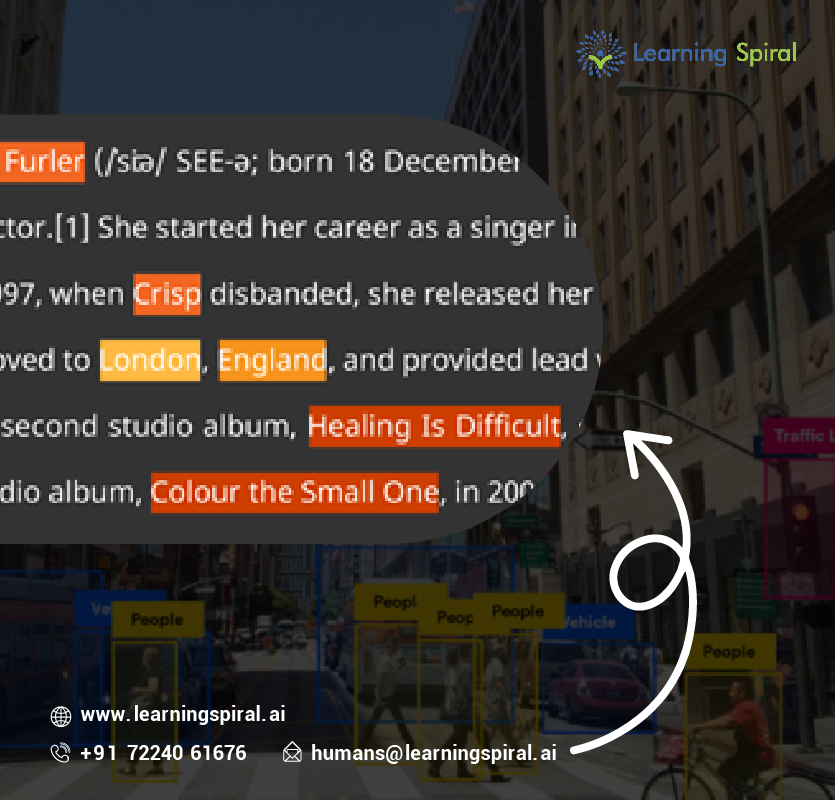
In the age of artificial intelligence, data is king. But raw data is meaningless to AI models – it needs to be labeled and organized for them to learn and perform tasks.
While image annotation often takes center stage, the world of data annotation extends far beyond pictures. Text annotation and more general data annotation play equally crucial roles in training AI models across various applications.
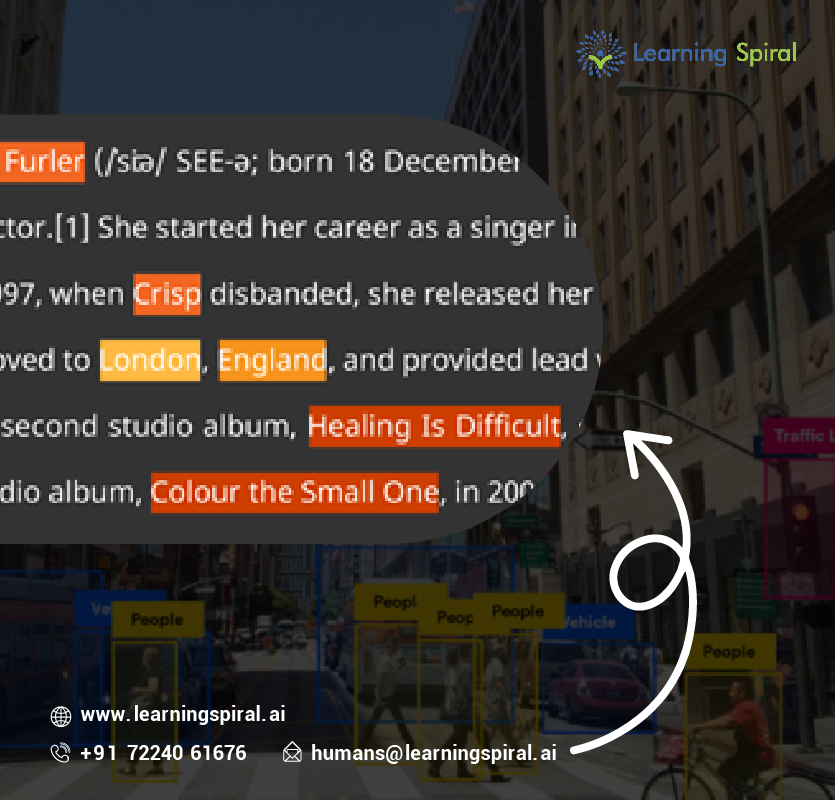
Text Annotation: The Power of Words
Text annotation involves enriching textual data with additional information. This can take various forms:
- Sentiment Analysis:
Annotators label text snippets as positive, negative, or neutral, allowing AI to understand the emotional tone of written content. This has applications in social media monitoring, customer service chatbots, and brand reputation analysis. - Named Entity Recognition (NER):
Here, annotators identify and classify specific entities within text, such as people, organizations, locations, and dates. This helps AI models extract key information from documents, emails, and news articles. - Intent Classification:
This involves labeling text based on the user’s intent behind the words. For example, in a customer service chat, an annotator might label a message as “request for refund” or “complaint about product.” This allows AI-powered chatbots to understand user needs and respond appropriately. - Part-of-Speech (POS) Tagging:
Annotators assign grammatical labels to each word (noun, verb, adjective) in a sentence. This helps AI models understand the structure and meaning of language, which is crucial for tasks like machine translation and text summarization.
Beyond Text: The Diverse Landscape of Data Annotation
Data annotation goes beyond text and images. Here are some other crucial areas:
- Speech Annotation:
Speech data is labeled with information like speaker identification, sentiment analysis, and transcription. This is essential for training AI assistants like Siri or Alexa to understand spoken language. - Sensor Data Annotation:
Data from sensors in various devices like wearables or self-driving cars needs to be labeled with context. For instance, sensor data from a smartwatch might be labeled as “walking” or “sleeping” based on user activity. - Time Series Data Annotation:
Data collected over time (e.g., stock prices, weather patterns) needs labeling to identify trends and anomalies.
The Benefits of Text and Data Annotation
Investing in text and data annotation offers several advantages:
- Improved AI Performance:
Accurate and well-labeled data leads to more accurate and reliable AI models. This is crucial for tasks where precision matters, such as medical diagnosis or financial fraud detection. - Enhanced User Experience:
AI applications trained on well-annotated data can provide a more natural and intuitive user experience. For instance, a chatbot with well-annotated training data can understand user queries more effectively. - Unlocking New Applications:
Text and data annotation open doors for a wider range of AI applications. By making diverse data understandable by AI, we can develop innovative solutions in areas like scientific research, environmental monitoring, and personalized learning.
Challenges and Considerations
Text and data annotation are not without their challenges:
- Data Bias: Annotated data can perpetuate existing biases if not carefully curated. It’s crucial to ensure a diverse pool of annotators and labeled data to avoid biased AI models.
- Cost and Time: Creating high-quality annotated datasets can be expensive and time-consuming. Exploring innovative labeling techniques and leveraging automation tools can help streamline the process.
- Data Security: Data annotation often involves sensitive information. Implementing robust security measures and adhering to data privacy regulations are essential.
The Future of Text and Data Annotation
As AI continues to evolve, the future of text and data annotation is bright:
- Automated Labeling Tools: The development of AI-powered tools can assist with repetitive tasks in the annotation process, improving efficiency and accuracy.
- Focus on Explainability: Developing AI models that can explain their reasoning behind decisions based on annotated data will foster trust and transparency in AI applications.
- Standardization of Annotation Practices: Standardized annotation guidelines and techniques will ensure consistency and improve the overall quality of labeled data.
Conclusion
Text and data annotation are the unsung heroes of the AI revolution. By unlocking the meaning within text, speech, and sensor data, they empower AI models to perform complex tasks and contribute to advancements across various industries. As we move forward, investing in responsible and ethical data annotation practices will be key to unlocking the full potential of AI for a better future.