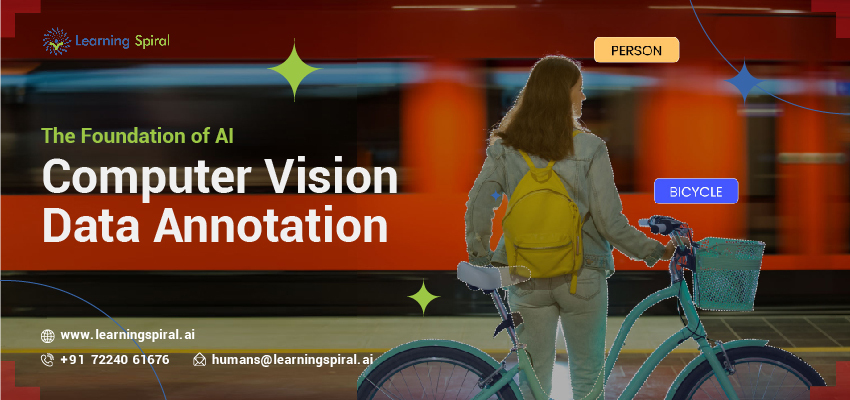
Computer vision, a field of artificial intelligence that enables computers to interpret and understand the visual world, relies heavily on high-quality data. This data is meticulously labeled or annotated to train machine learning models.
The object behind doing so is guiding them to recognize patterns, objects, and scenes within images and videos. This process is known as computer vision data annotation.
The Importance of Accurate Annotation
Accurate annotation is crucial for the success of computer vision systems. It provides the models with the necessary information to learn and make informed decisions. For example, in object detection, annotators might outline the boundaries of objects within images, helping the model to identify and localize them.
In image segmentation, annotators might segment images into different regions or classes, teaching the model to understand the composition of scenes.
Types of Computer Vision Data Annotation
There are several common types of computer vision data annotation:
- Object Detection: Involves drawing bounding boxes around objects within images or videos, specifying their location and size.
- Image Segmentation: Requires annotators to delineate regions or objects within images, creating pixel-level masks that define their boundaries.
- Landmark Detection: Involves marking specific points or landmarks within images, such as facial keypoints or object corners.
- Image Classification: Involves assigning class labels to entire images, indicating the overall content or category.
- Semantic Segmentation: Similar to image segmentation but at a finer level, assigning semantic labels to each pixel in an image, indicating the object or class it belongs to.
- Instance Segmentation: A combination of object detection and semantic segmentation, identifying and segmenting individual instances of objects within an image.
Challenges and Best Practices
Computer vision data annotation can be a time-consuming and labor-intensive process, especially for complex datasets. Some of the challenges include:
- Data Quality: Ensuring the accuracy and consistency of annotations is critical for training effective models.
- Label Complexity: Some tasks, such as instance segmentation or fine-grained object recognition, require highly detailed and precise annotations.
- Data Volume: Large-scale datasets are often necessary for training robust models, but acquiring and annotating such data can be challenging.
To address these challenges, organizations often employ a combination of human annotators and automated tools. Human annotators can provide expert judgment and handle complex cases, while automated tools can streamline the process and improve efficiency.
Additionally, best practices such as clear guidelines, quality control measures, and version control can help ensure the quality and consistency of annotated data.
Applications of Computer Vision Data Annotation
Computer vision data annotation has a wide range of applications across various industries, including:
- Autonomous Vehicles: Training self-driving cars to recognize objects, pedestrians, and traffic signs.
- Medical Imaging: Analyzing medical images for diagnosis and treatment planning.
- Facial Recognition: Developing systems for identifying and verifying individuals.
- Retail: Enhancing customer experiences through visual search and product recommendations.
- Manufacturing: Improving quality control and automation through visual inspection.
As computer vision technology continues to advance, the demand for high-quality annotated data will only grow. By understanding the principles of computer vision data annotation and addressing the associated challenges, organizations can develop powerful and accurate AI systems.