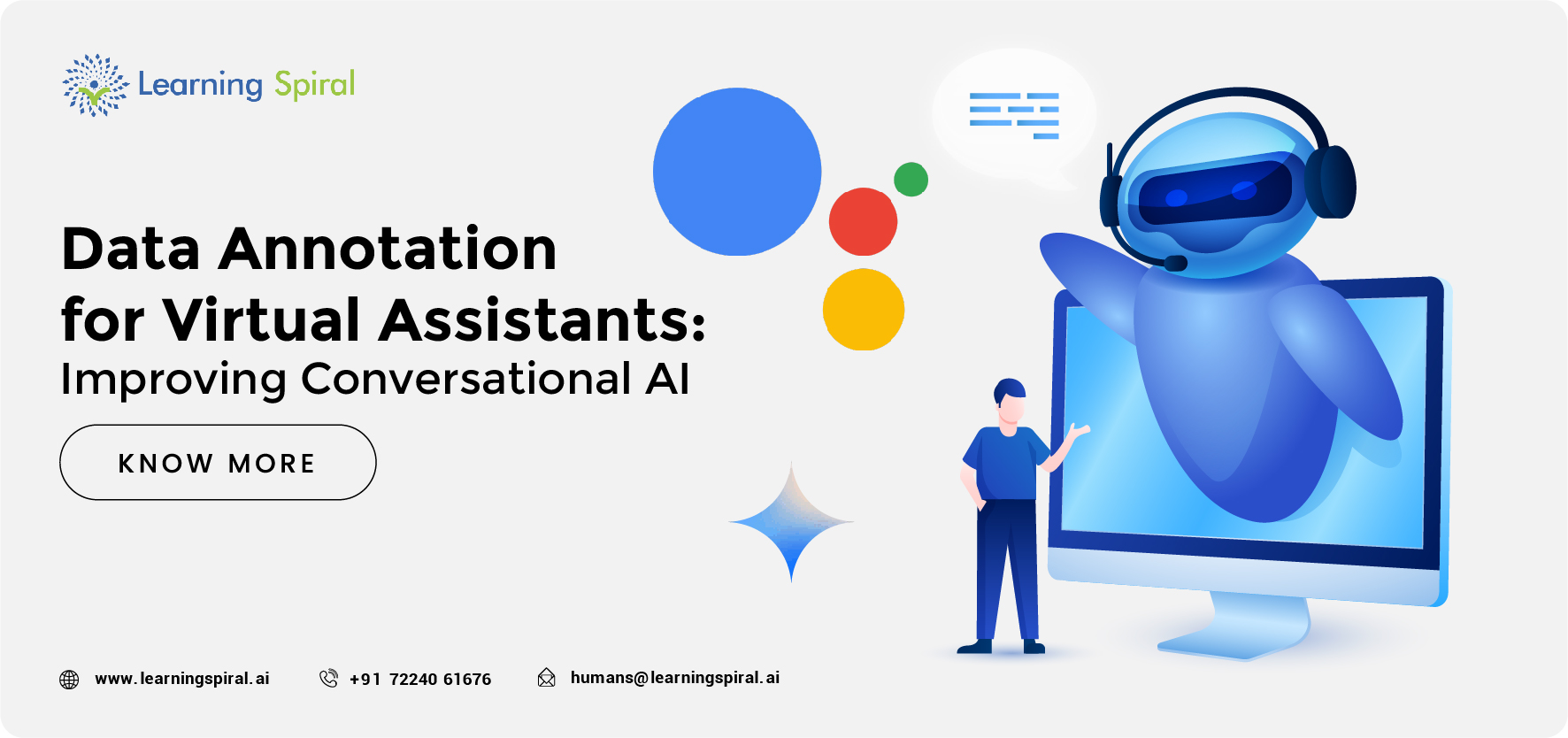
Virtual assistants, usually present as customer support bots by artificial intelligence on websites, have become increasingly familiar in our lives. Aside from assisting visitors on sites or answering queries, these assistants help set alarms and control smart home devices.
These AI-powered companions rely on a crucial element for success: high-quality data annotation.
Data annotation acts as the bridge between raw data and a virtual assistant’s ability to understand and respond to human communication. Just like a child learning a language, virtual assistants need vast amounts of labeled data to grasp the nuances of human conversation.
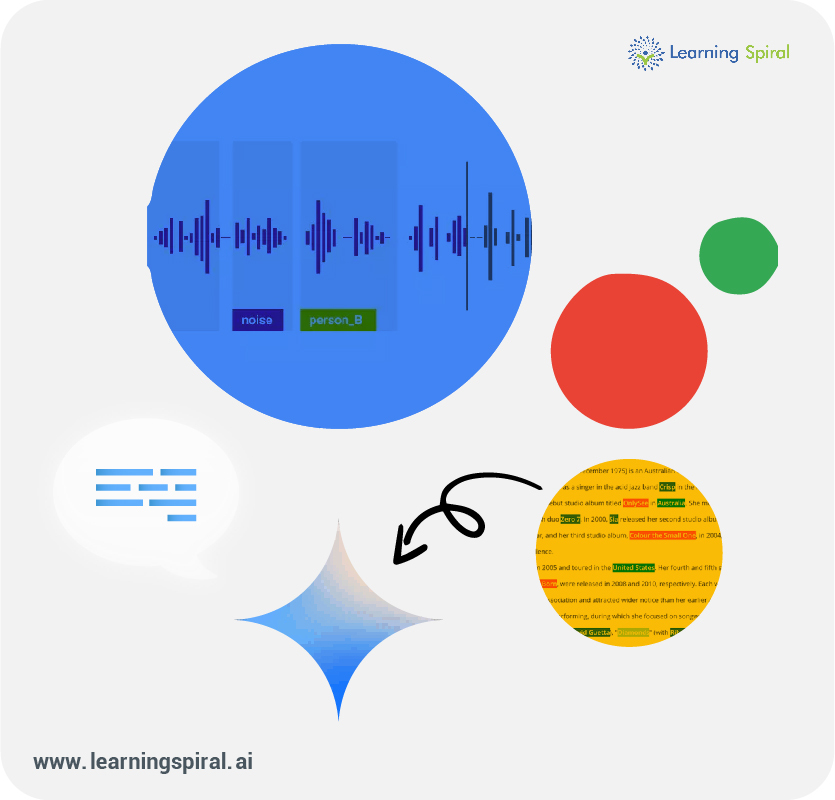
Hence, the data annotation process unlocks the potential of conversational AI, paving the way for more natural and effective interactions.
Understanding the Importance of this Partnership
Conversational AI thrives on three key components within data annotation:
- Entities:
These are the specific details mentioned in user queries. Annotating entities, like locations, names, or dates, helps virtual assistants identify what information users are referring to.
- Intents:
Intents represent the underlying goal behind a user’s utterance. Is a user asking a question, requesting a service, or issuing a command? Recognizing intent allows virtual assistants to respond appropriately.
- Context:
Conversations aren’t isolated events. Understanding the broader context of a conversation allows virtual assistants to maintain a natural flow and avoid misinterpretations.
The Power of Precise Data Annotation
Precise data annotation empowers virtual assistants in several ways:
- Enhanced Accuracy: By identifying entities and intents accurately, virtual assistants can deliver more relevant and helpful responses, reducing user frustration and improving satisfaction.
- Natural Language Processing: Data annotation fuels the ability of virtual assistants to process the complexities of human language, including slang, sarcasm, and colloquialisms. This fosters a more natural and engaging user experience.
- Personalized Interactions: Annotated data can be tailored to specific domains or user demographics. This allows virtual assistants to cater their responses to individual needs and preferences.
Challenges and Considerations
While data annotation holds immense potential, there are challenges to consider:
- Data Volume: Training effective virtual assistants requires vast amounts of annotated data. Gathering and processing this data can be a significant undertaking.
- Data Quality: The quality of data annotation directly impacts the performance of virtual assistants. Inaccurate or inconsistent annotations lead to misunderstandings and hinder effectiveness.
- Privacy Concerns: Data annotation often invo[[olves human interaction with user queries, which can raise privacy concerns. Ensuring robust security measures is paramount.
Conclusion
Data annotation serves as the lifeblood of successful virtual assistants. By providing virtual assistants with the ability to understand the complexities of human communication, data annotation paves the way for more natural, accurate, and personalized interactions. As data annotation techniques continue to evolve, we can expect virtual assistants to become even more integrated and indispensable parts of our lives.