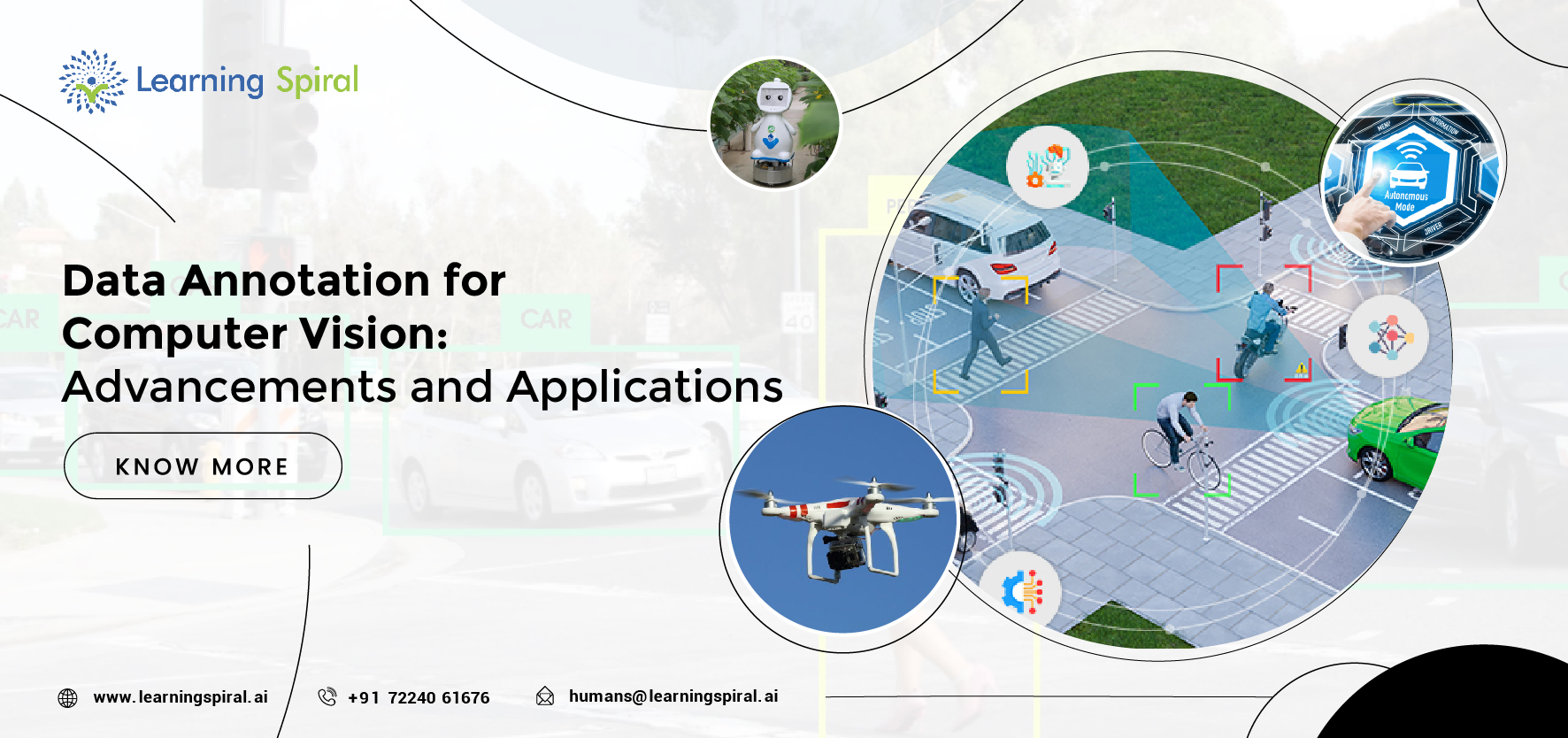
The world of computer vision is rapidly evolving, fueled by ever-growing datasets and sophisticated algorithms. But behind these advancements lies a crucial process: data annotation. Labeling data in an image, text, video, or audio to help AI and machine learning models analyze the right content and generate appropriate solutions is the base for computer vision.
Annotating visual data for training computer vision models requires meticulous labeling and categorization, effectively teaching machines to “see” and understand the world around them. Before we get to the advancements in the field, let’s first figure out what changes have been made in the past few years.
Traditional vs. Advanced Techniques:
Traditionally, data annotation involved manual efforts by human annotators. They’d painstakingly draw bounding boxes around objects, classify images, and identify intricate details. While effective, this process was often time-consuming, expensive, and prone to human error.
Recent advancements have revolutionized data annotation, introducing:
- Active Learning: Algorithms prioritize annotating data points that bring the most value to the model, optimizing efficiency.
- Semi-Supervised Learning: Unlabeled data is leveraged alongside smaller amounts of labeled data for model training, reducing annotation requirements.
- Weak Supervision: Annotators provide less granular labels (e.g., “outdoor scene”) focusing on broader concepts, often faster and more scalable.
- Transfer Learning: Pre-trained models on existing datasets can be adapted for new tasks, requiring less training data and annotation.
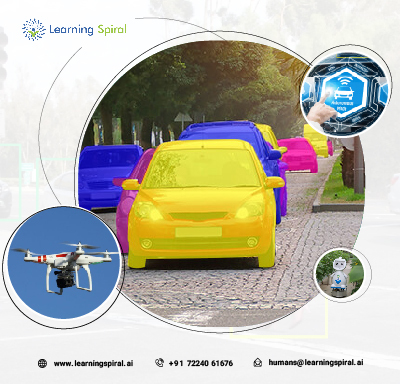
Applications Across Industries:
Computer vision, fueled by high-quality annotated data, is transforming diverse industries:
- Healthcare: AI-powered medical imaging analysis detects diseases like cancer with greater accuracy and speed, aiding early diagnosis and treatment.
- Autonomous Vehicles: Self-driving cars rely on annotated data to identify objects, navigate roads, and make critical decisions in real-time.
- Retail: Image recognition powers product searches, personalized recommendations, and automated inventory management, enhancing customer experience.
- Security and Surveillance: Facial recognition and anomaly detection systems, trained on labeled video data, improve security measures and prevent crime.
- Agriculture: Drones equipped with computer vision technology analyze crop health, optimize resource usage, and automate tasks, promoting sustainable farming practices.
Emerging Trends and Challenges:
The future of data annotation holds exciting possibilities:
- 3D Data Annotation: Annotating point clouds and 3D models will unlock advanced applications in robotics, AR/VR, and autonomous systems.
- Collaborative Annotation Platforms: Democratizing data annotation through secure platforms with diverse annotator communities will accelerate data labeling capabilities.
- Explainable AI: Understanding how computer vision models make decisions based on annotated data will enhance trust and transparency in their applications.
However, challenges remain:
- Bias and Fairness: Ensuring diverse and representative datasets in annotation is crucial to avoid biased AI models.
- Privacy Concerns: Balancing the need for data with individual privacy and ethical considerations is crucial.
- Continuous Learning: Adapting annotation strategies to evolving real-world scenarios and emerging technologies is essential for lasting impact.
Conclusion:
Data annotation, though often invisible, forms the backbone of computer vision advancements. By embracing innovative techniques, exploring diverse applications, and addressing emerging challenges, we can unlock the full potential of this technology to shape a smarter, safer, and more efficient future.