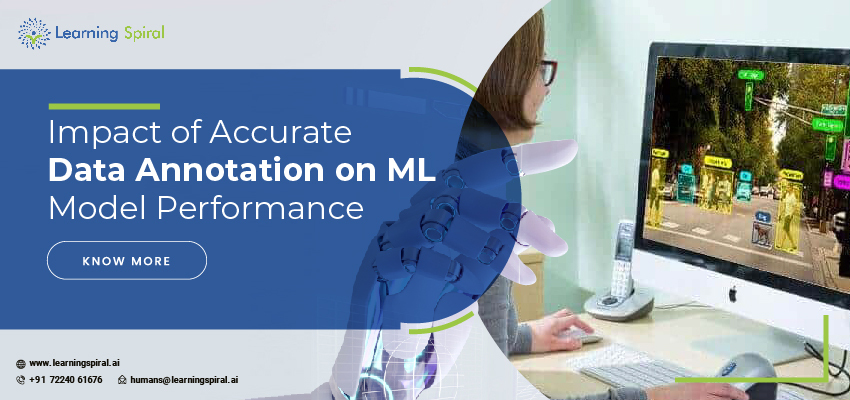
The success of any Machine Learning aka ML model hinges on the quality of its training data. Accurate data annotation, where humans or AI interpret and label data for algorithms, plays a pivotal role in shaping model performance.
Let’s delve into four diverse case studies to illustrate how meticulous data annotation translates into real-world impact:
1. Facial Recognition: Unveiling Identity with Precision
Facial recognition technology relies heavily on precise data annotation. Annotators meticulously label facial features, landmarks, and expressions in massive image datasets. This allows models to learn subtle variations in lighting, pose, and age, leading to superior recognition accuracy.
In security applications, accurate facial recognition systems can identify wanted individuals with minimal false positives, enhancing public safety. For personalized experiences, accurate annotation enables retailers to offer targeted recommendations based on customer demographics, boosting engagement and sales.
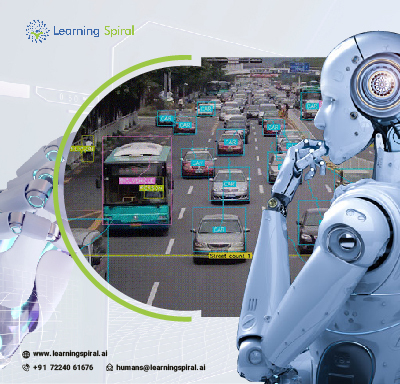
2. Self-Driving Cars: Navigating the Road with Confidence
Autonomous vehicles require training data that matches the complexities of real-world driving scenarios. Annotators accurately label objects like pedestrians, vehicles, traffic signs, and lane markings in images and video footage.
This enables self-driving cars to make accurate decisions while navigating traffic, obeying rules, and avoiding obstacles. Accurate annotation of diverse weather conditions and edge cases further strengthens performance, promoting safer and more reliable autonomous driving experiences.
3. Drones & Satellites: Seeing Beyond Human Scope
Aerial data captured by drones and satellites provides valuable insights for environmental monitoring, disaster management, and agricultural analytics. Annotators identify land-use patterns, vegetation types, and infrastructure damage in aerial images, empowering users to track deforestation, predict crop yields, and respond effectively to natural disasters.
The accuracy of these annotations directly impacts the quality of information extracted, leading to data-driven decision-making and improved resource management.
4. Figure Detection: Unveiling the Unseen in Medical Images
Medical imaging technologies like X-rays and CT scans play a crucial role in early disease detection and diagnosis. Annotators meticulously label tumors, fractures, and other abnormalities in these images, allowing AI models to identify subtle patterns invisible to the human eye.
Accurate figure detection in medical images leads to faster and more accurate diagnoses, personalized treatment plans, and improved patient outcomes. The impact of precise annotation in this field resonates profoundly, saving lives and promoting better healthcare.
Conclusion
These case studies showcase the significant impact of accurate data annotation on the performance of machine learning models. Investing in meticulous annotation processes not only enhances model accuracy, but also unlocks real-world applications that improve safety, efficiency, and decision-making across diverse industries.
As technology advances, the role of data annotation will become even more crucial in shaping the future of AI-powered solutions that benefit humanity in myriad ways.