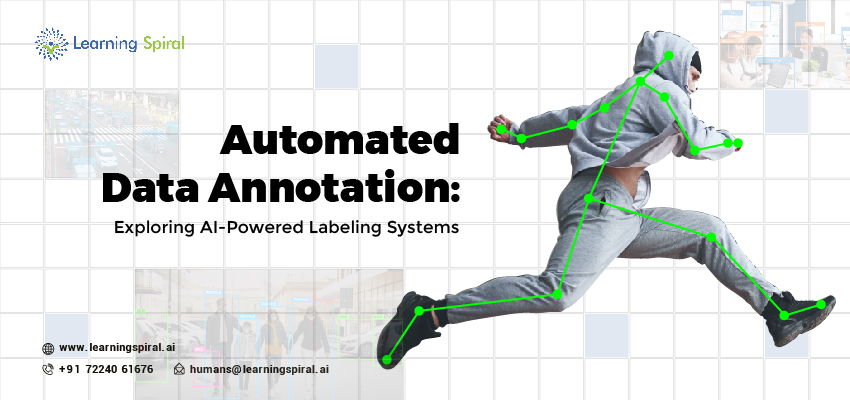
Everything related to data comes with varying degrees of complications, especially when the data is being utilized for annotation. The demand for high-quality labeled data is ever-growing.
There are two ways to annotate or label data. One is via manual sources, and the other is automation. However, manually annotating vast datasets can be time-consuming, expensive, and prone to human error. This is where automated data annotation enters the scene. By leveraging the power of AI, the labeling process is streamlined and revolutionize, allowing accurate training of machine learning models.
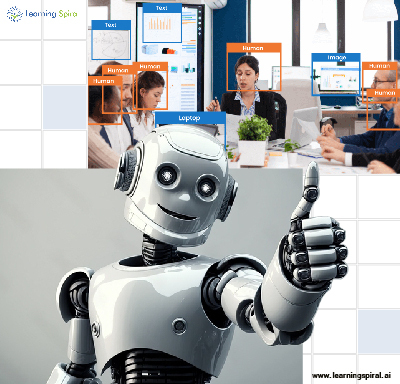
Manual vs. Automated Annotation:
Traditionally, data annotation relies on human effort, where teams meticulously label images, videos, and text by expert standards. While effective, this approach suffers from:
- High Cost: Human labor is expensive, and large datasets require significant resources.
- Slow & Scalability Issues: Manual annotation is time-consuming and struggles to scale with growing data volumes.
- Subjectivity and Error: Human annotators are prone to errors and inconsistency, introducing bias and impacting model performance.
AI-powered systems aka automated annotation address these limitations by automating various aspects of data annotation. Here are some key techniques:
- Pre-annotation: AI suggests labels based on existing data, drastically reducing manual effort.
- Active learning: The system selects data points with the highest impact on model learning, prioritizing annotation effort.
- Weak supervision: Labels are less granular (e.g., “outdoor scene”) but faster to provide, guiding the model’s understanding.
- Supervised learning: Existing labeled data is used to train a model that then annotates new data automatically.
Benefits and Applications of Automated Annotation:
Automated data annotation offers several advantages. Some of which are:
- Reduced Costs: Saves time and resources compared to manual annotation, making large-scale data preparation more feasible.
- Increased Efficiency: Automates repetitive tasks, allowing human annotators to focus on complex cases.
- Improved Scalability: Handles growing data volumes with ease, supporting larger and more ambitious projects.
- Reduced Bias: Can objectively apply labeling rules, minimizing subjective biases inherent in human annotation.
These benefits allows a myriad of applications across diverse fields to open route for data annotation:
- Computer vision: Automating object detection, image classification, and scene understanding for self-driving cars, medical imaging, and robotics.
- Natural language processing: Annotating text data for sentiment analysis, topic extraction, and machine translation.
- Speech recognition: Labeling audio data for automated transcription and voice assistants.
Challenges:
While promising, every aspect of automated data annotation doesn’t come with a silver lining. Some of the major challenges that befall while using this method are:
- AI Model Performance: The accuracy of automated annotations depends on the underlying AI model, requiring careful selection and training.
- Explainability and Trust: Understanding how AI models arrive at labels is crucial for building trust and identifying potential biases.
- Limited Scope: Certain tasks still require human expertise, and ethical considerations regarding data privacy and fairness remain paramount.
How to Reduce the Uprising Challenges in Automated Data Annotation?
Automated data annotation is rapidly evolving, driven by advancements in AI and machine learning. We can expect:
- More Sophisticated AI Models: Improved accuracy, explainability, and ability to handle complex tasks.
- Domain-Specific Solutions: Systems tailored to specific industries and applications.
- Hybrid Approaches: Combining human expertise with AI automation for optimal results.
Conclusion:
Automated data annotation holds immense potential to revolutionize data-driven development. By understanding its capabilities, limitations, and ethical considerations, we can harness this technology. Hence, unlocking the true potential of AI, accelerating innovation and progress in diverse fields.