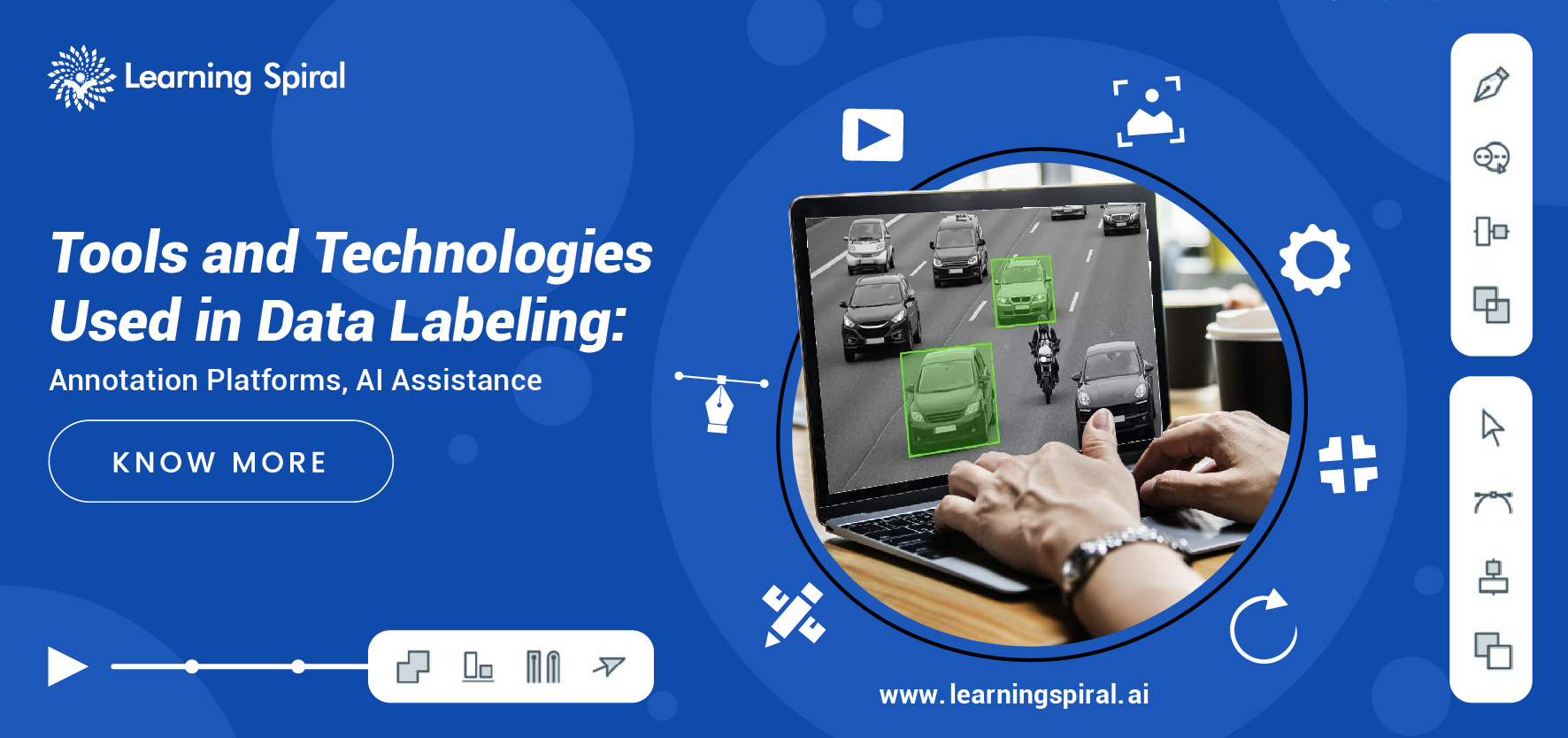
Data labeling, the meticulous process of tagging data with relevant information, forms the backbone of training powerful AI models. However, the sheer volume and complexity of data used today necessitates sophisticated tools and technologies to streamline and enhance the labeling process.
This article delves into the key tools and explores the emerging role of AI in assisting human labelers.
Annotation Platforms: The Foundation of Labeling
Annotation platforms serve as the central hub for all data labeling activities. These web-based platforms provide a user-friendly interface for labelers to access, interact with, and tag various data types. Here are some key features of annotation platforms:
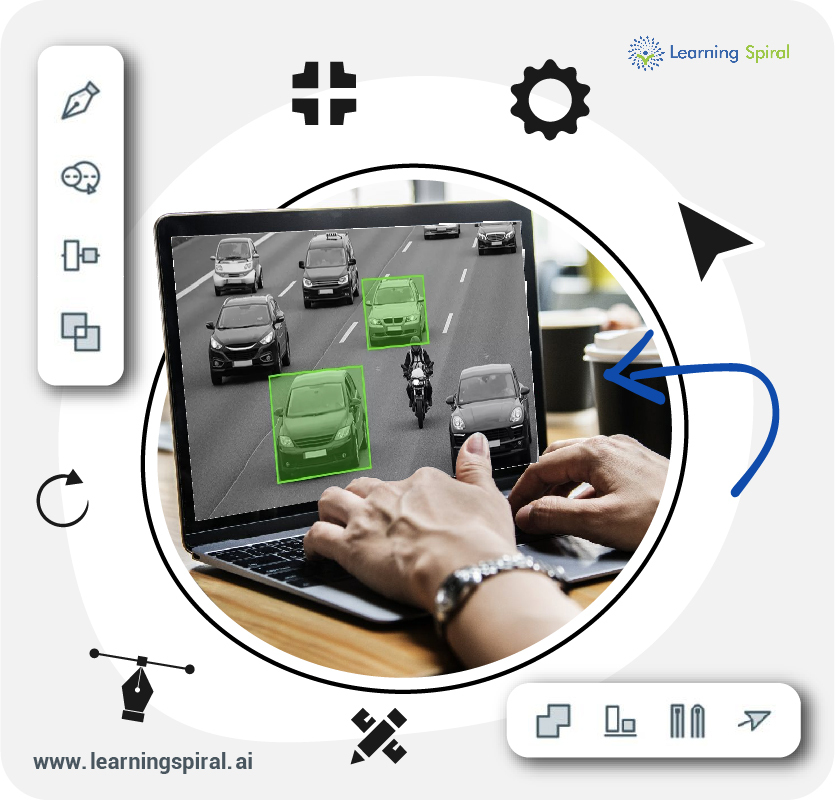
- Data Upload and Management:
Platforms offer seamless upload options for different data formats – images, videos, text, and more. They also enable efficient data organization with features like tagging, filtering, and search functionalities.
- Annotation Tools:
The core functionality lies in the diverse annotation tools offered. These tools allow for various labeling tasks like bounding boxes for object detection, polygon creation for image segmentation, and sentiment labeling for text data.
- Collaboration Features:
Many platforms facilitate collaboration between labelers, enabling them to work on projects simultaneously and share annotations for consistency. Features like chat tools and task assignment further streamline workflows.
- Quality Control Mechanisms:
Data quality is paramount. Platforms often incorporate built-in quality checks, like label validation and inter-annotator agreement scores, to ensure accuracy and consistency in labeling.
- Customization and Automation:
Advanced platforms offer customization options to tailor the labeling interface and workflows to specific project needs. Some platforms even provide basic automation features to expedite repetitive tasks.
The Rise of AI-Assisted Labeling
While annotation platforms empower human labelers, the sheer volume of data can lead to bottlenecks. This is where AI is stepping in to revolutionize data labeling:
- Active Learning: This technique leverages a pre-trained model to identify data points with the highest uncertainty. These are then prioritized for human labeling, maximizing labeling efficiency.
- Auto-labeling: AI models can be trained to perform basic labeling tasks for simpler datasets. This frees up human labelers to focus on complex tasks requiring nuance and judgment.
- Semi-supervised Learning: This approach utilizes a combination of labeled and unlabeled data. AI models can learn from the labeled data and then propose labels for the unlabeled data, which human experts can then validate or refine.
The Future of Data Labeling: A Symbiotic Partnership
The future of data labeling lies in a harmonious collaboration between human expertise and AI capabilities. Here’s what we can expect:
- Smarter AI Assistants: AI models will become adept at identifying labeling errors, suggesting corrections, and even pre-filling labels for specific data points.
- Human-in-the-Loop Workflows: The focus will shift towards leveraging AI for repetitive tasks while reserving human expertise for complex judgment and nuanced labeling decisions.
- Explainable AI: Transparency in AI-assisted labeling will be crucial. Explainable AI techniques will help human labelers understand the rationale behind AI suggestions, fostering trust and collaboration.
Conclusion
Data labeling tools and AI assistance are revolutionizing the way we prepare data for AI models. By leveraging these advancements, organizations can ensure efficient, accurate, and scalable data labeling, ultimately paving the way for the development of more robust and reliable AI applications.
As the field continues to evolve, the synergy between human intelligence and AI will be the key to unlocking the full potential of data labeling for the future of AI.