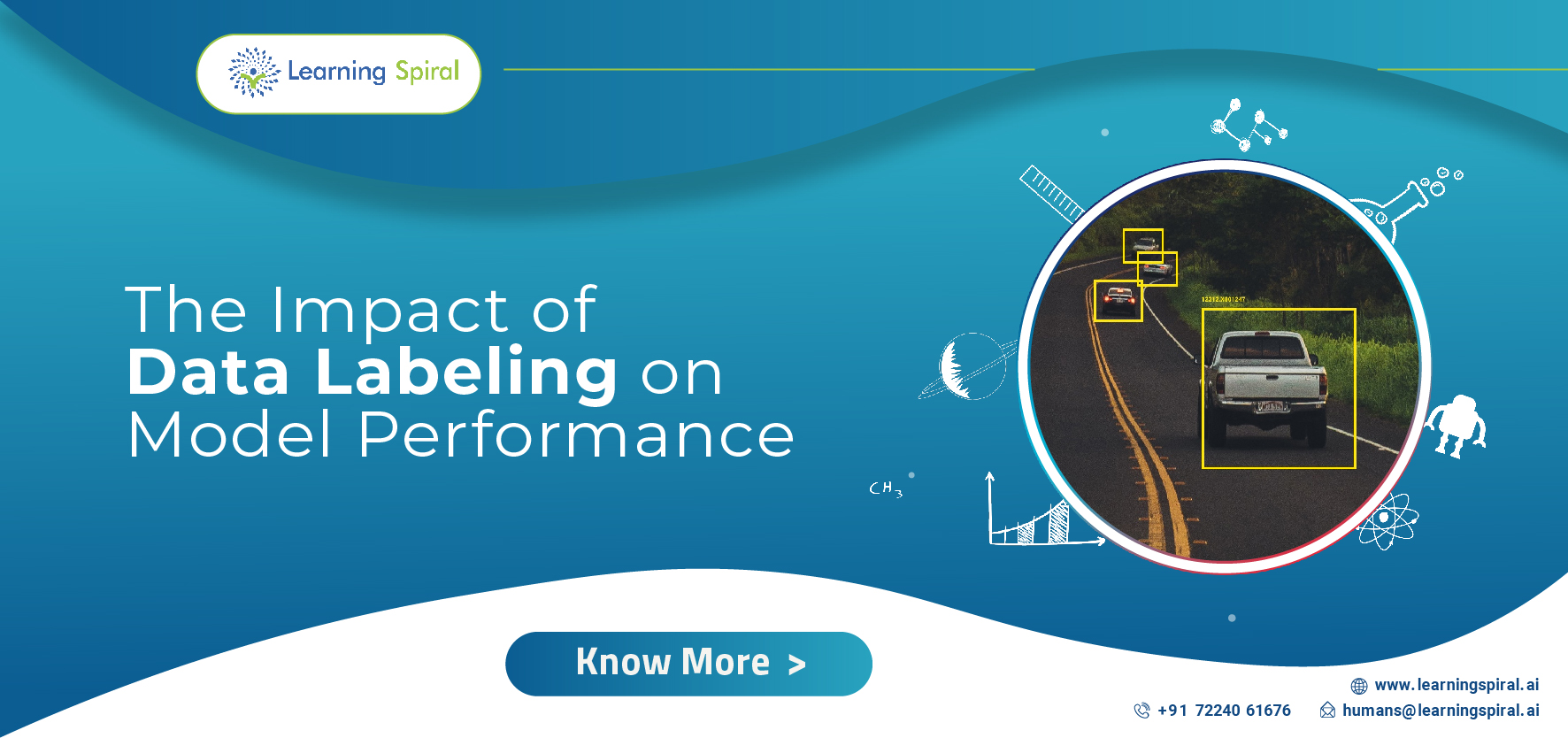
Data labeling is the process of assigning labels to data points. This is an essential step in the machine learning process, as it helps the model to learn and make predictions. Hence data labeling services boost AI development in the world. The quality of the data labeling process can have a significant impact on the performance of the model being labeled.
If the data labeling is inaccurate or incomplete, the model will not be able to learn as effectively. This can lead to poor performance, such as inaccurate predictions or low authenticity. In some cases, it can even lead to the model making decisions leading to dangerous or harmful outcomes.
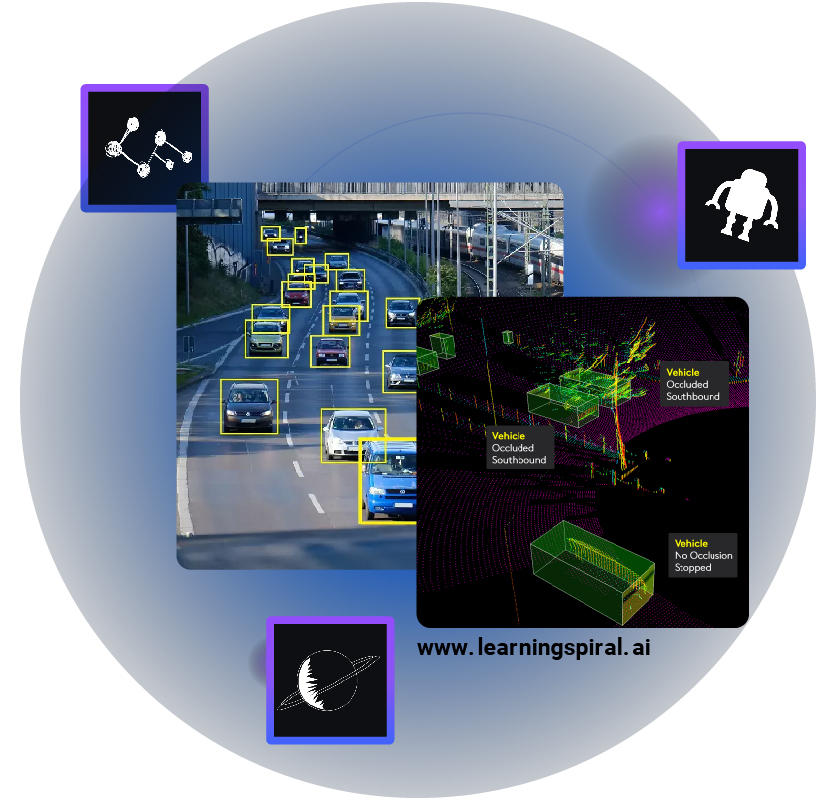
For example, a model that is used to classify images of medical conditions needs to be trained on a dataset of accurately labeled images. If the labels are inaccurate, the model may not be able to distinguish between different conditions. This could lead to a doctor misdiagnosing a patient, which could have serious consequences.
The impact of data labeling on model performance can be seen in a number of different areas, including:
- Accuracy: The accuracy of a model highly makes up a large part of an effective data labeling service company as it is directly related to machines making the correct prediction.
- Recall: Recall is the percentage of positive examples that are correctly identified by the model. A model with accurate data labeling will typically have a higher recall than a model with inaccurate data labeling.
- Precision: Precision is the percentage of predicted positive examples that are actually positive. A model with accurate data labeling will typically have higher precision than a model with inaccurate data labeling.
In addition to accuracy, recall, and precision, data labeling can also impact other aspects of model performance, such as the speed of the model and the cost of training the model. To learn about the in-depth ins and outs of data labeling services, you can check out one of the best data labeling companies in the market today, Learning Spiral.