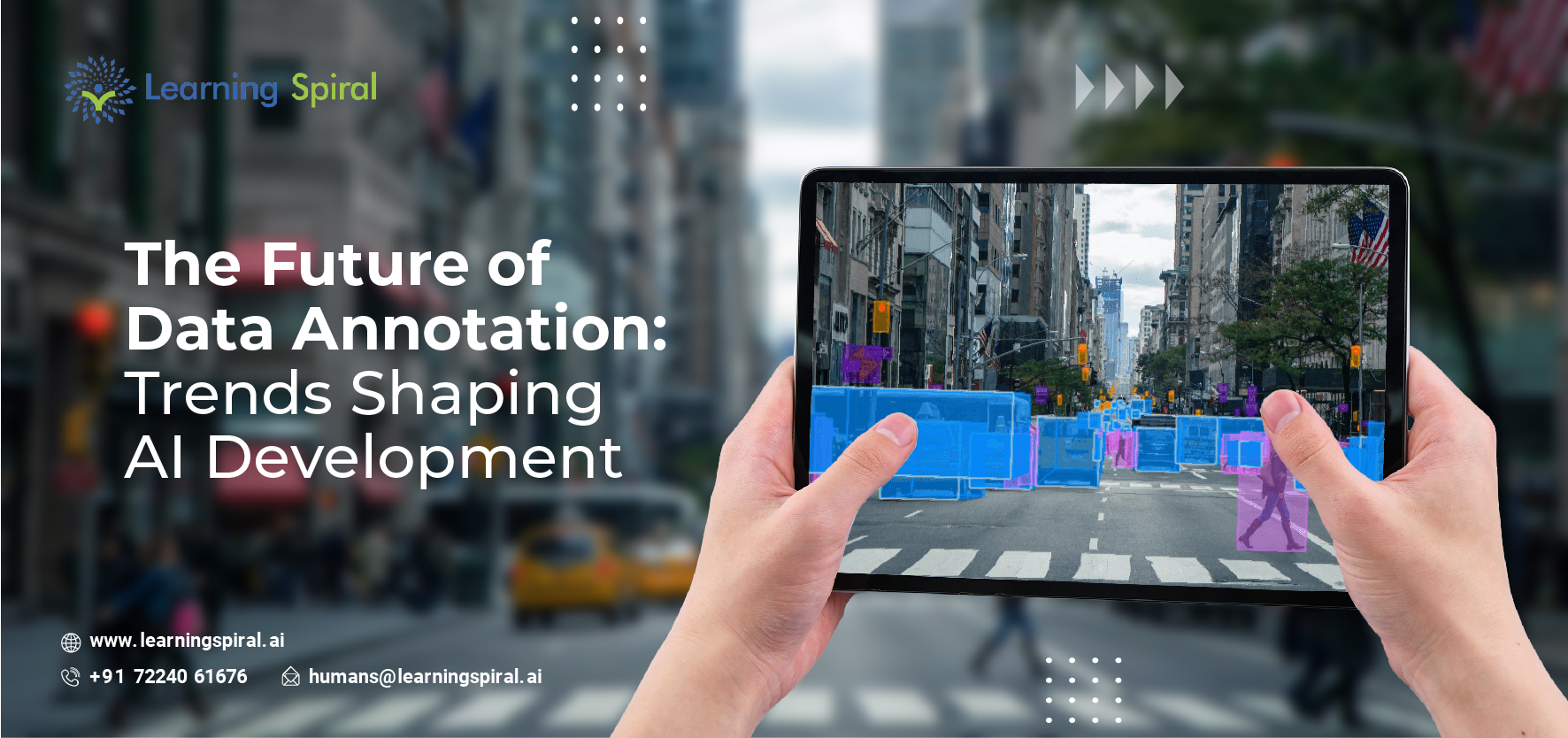
Data annotation, the process of tagging (labeling) and interpreting data for AI algorithms, has long been considered the silent engine driving the AI revolution. But as AI aspirations reach new heights, the world of data annotation is itself undergoing a paradigm shift.
Let’s explore the emerging trends shaping the future of data annotation and their impact on AI development:
1. Automation Ascends:
The manual, time-consuming nature of traditional annotation is giving way to AI-powered automation. Active learning algorithms can autonomously suggest which data points require human annotation, prioritizing efficiency and cost reduction.
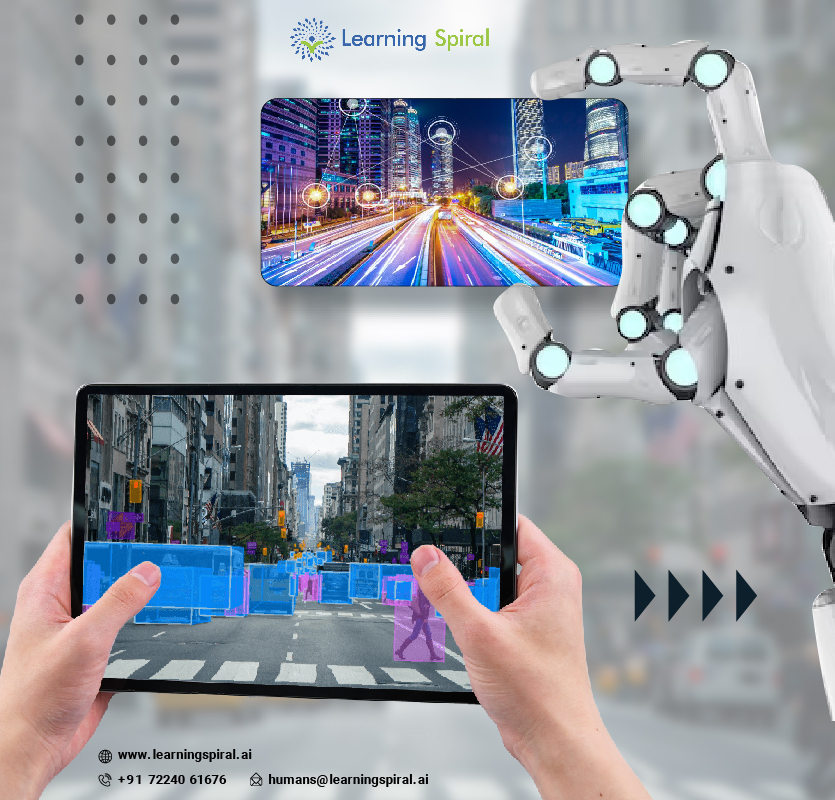
For repetitive tasks like image tagging, semi-automated tools can pre-annotate data, leaving human experts to focus on nuanced annotations.
2. Beyond Boxes and Tags:
Data annotation is moving beyond simple object tagging in images to encompass complex tasks like semantic segmentation, sentiment analysis, and relationship extraction. This necessitates more sophisticated annotation tools that handle intricate data types like audio, text, and sensor data.
Advanced annotation platforms will become central to handling the diverse and dynamic nature of AI datasets.
3. Democratization of Annotation:
Traditionally, high-quality data annotation required specialized expertise, limiting access to smaller startups and researchers. With the rise of collaborative annotation platforms and crowd-sourcing models, even smaller players can access high-quality annotated data.
This democratization fosters broader innovation and accelerates the development of diverse AI applications.
4. Ethical Considerations:
As AI becomes more prevalent, responsible data annotation practices become crucial. Concerns around bias, data privacy, and worker well-being are pushing for greater transparency and ethical sourcing of annotated data.
Fair compensation for annotators, diverse annotation teams, and robust data anonymization are key factors in building trustworthy AI solutions.
5. The Rise of Synthetic Data:
To address the ever-growing demand for realistic training data, synthetic data generation tools are emerging. These tools can create artificial images, videos, and text data, reducing reliance on real-world data collection and mitigating privacy concerns.
The integration of synthetic data into annotation workflows will further optimize AI model training and performance.
These trends illustrate the evolving landscape of data annotation. It’s no longer just about labeling data; it’s about building a robust, ethical, and efficient ecosystem that fuels the next generation of AI advancements. As these trends converge, we can expect AI to become more nuanced, inclusive, and capable of tackling real-world challenges across diverse domains.
Ultimately, the future of data annotation promises to be an exciting space where human ingenuity and technological innovation pave the way for a smarter, more just future powered by AI.