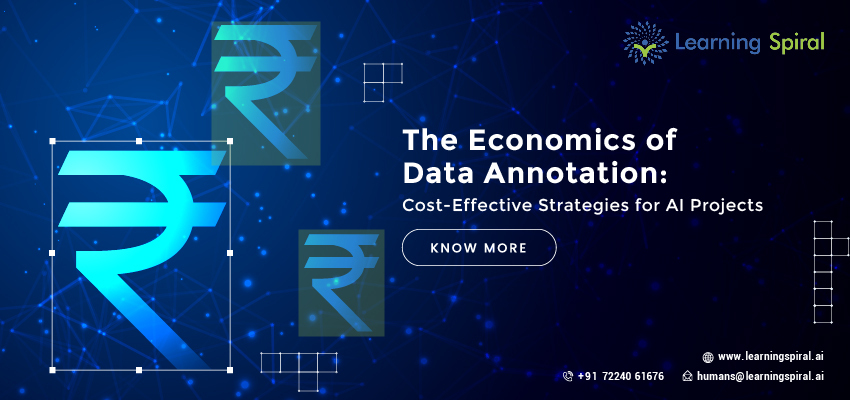
In the rapidly advancing field of AI, data reigns supreme. But data alone isn’t enough; high-quality, meticulously labeled, and accurate data holds the key to unlocking the true potential of machine learning models.
However, acquiring and annotating massive datasets can be a financial hurdle, especially for startups and smaller organizations. Navigating the economics of data annotation becomes crucial for balancing cost-effectiveness with achieving desired model performance.
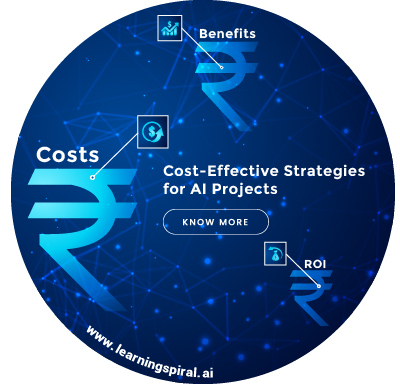
Here, we will explore strategies to optimize your approach and build successful AI projects without breaking the bank.
Understanding the Cost Drivers:
Before we get into managing the costs, let’s unravel what are the areas extracting high costs under data annotation.
- Images, videos, and audio require more intricate labeling compared to text data, impacting annotation time and cost.
- Larger datasets translate to more data points to annotate, directly affecting overall cost.
- Different kinds of annotations and labeling have different attention spans, and prices.
- In-house teams might incur recruitment, training, and management costs, while outsourcing options have their own pricing structures.
Strategies for Cost Optimization:
While cost-cutting is tempting, one cannot play with quality as it can undermine the entire project. Inaccurate or inconsistent labels lead to poor model performance, requiring retracing steps or rebuilding models, ultimately costing more in the long run.
- Prioritize Data Quality: Focus on gathering high-quality data relevant to your specific task. Avoid collecting unnecessary data as it adds to annotation costs without contributing to performance.
- Clearly Define Annotation Needs: Determine the precise level of detail required for your labels. Simpler annotations might suffice for some tasks, reducing complexity and cost.
- Explore Semi-supervised Learning: Utilize unlabeled data alongside smaller amounts of labeled data, potentially reducing the overall annotation burden.
- Consider Transfer Learning: Pre-trained models on massive datasets can be fine-tuned for your specific task, requiring less training data and annotation.
- Evaluate In-house vs. Outsourcing: Weigh the pros and cons of building an internal team with external annotation services. Consider factors like scalability, expertise, and cost structure.
- Utilize Crowdsourcing Platforms: These platforms offer access to large pools of annotators, but ensuring quality control and data security requires careful management.
- Automate Repetitive Tasks: Leverage annotation tools for tasks like pre-filling labels or automating simple checks, freeing up human annotators for complex tasks.
Beyond Cost:
While cost minimization is crucial, remember that successful AI projects go beyond just the price tag. Consider these additional factors:
- Time to Market: Faster annotation cycles through efficient strategies can expedite project completion and time-to-market advantages.
- Ethical Considerations: Prioritize fair and unbiased data annotation practices to ensure responsible AI development.
- Long-Term Scalability: Choose solutions that can scale with your evolving data needs and project growth.
Conclusion:
By understanding the cost drivers, employing strategic techniques, and considering factors beyond pure cost, one can optimize the data annotation process and build successful AI projects at cost effective strategies.
Remember, cost-effectiveness isn’t just about saving money; it’s about making smart investments that maximize the value and impact of your AI endeavors.