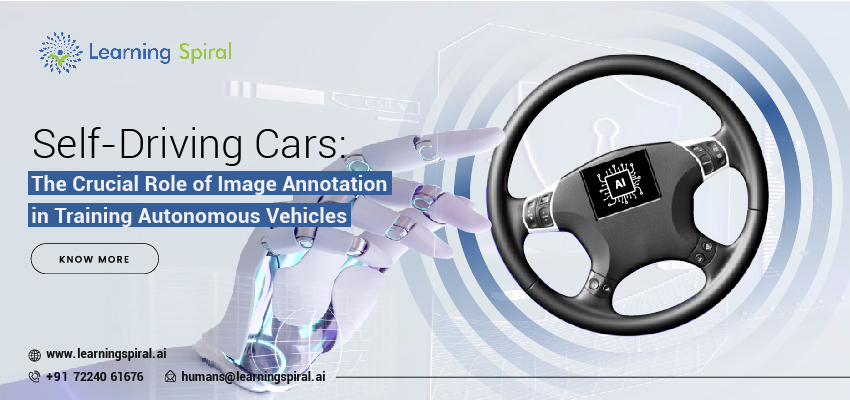
The vision of self-driving cars navigating city streets seamlessly is no longer science fiction. However, the journey towards fully autonomous vehicles requires significant advancements in Artificial Intelligence (AI), particularly in the area of computer vision.
Here’s where image annotation steps in as a critical player in training the brains of self-driving cars.
The Power of Image Annotation
Self-driving cars rely on complex algorithms that perceive and interpret their surroundings. This perception comes primarily from cameras mounted on the vehicle, capturing real-time video data.
To train these algorithms to ‘see’ and understand the world like a human driver, they need vast amounts of labeled image data. This is where image annotation comes into play.
First Step of The Annotation Process: Teaching the Car to See
Image annotation involves meticulously labeling images with specific information. In the context of self-driving cars, this might include:
- Object Detection: Labeling and identifying objects in the image, such as pedestrians, vehicles, traffic signs, and lanes.
- Bounding Boxes: Drawing boxes around each object to define its location and size.
- Attribute Labeling: Assigning additional attributes to objects, like the color of a traffic light or the direction a pedestrian is facing.
Lane Marking: Labeling the boundaries of lanes on the road.
By providing high-quality labeled images, data annotators essentially teach the AI system what different objects are and how to react to them in various situations. The more diverse and meticulously labeled the data, the better the AI model can understand the complexities of the real world.
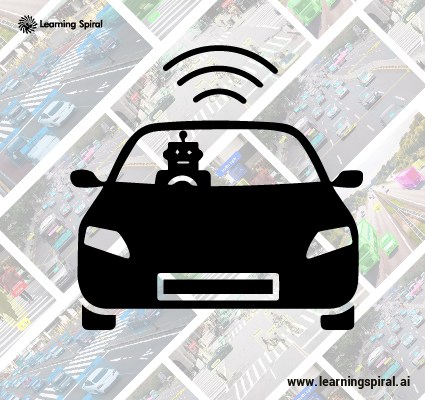
The Benefits of High-Quality Image Annotation
Investing in high-quality image annotation offers several advantages for self-driving car development:
- Improved Object Recognition: Accurate labeling ensures the AI accurately recognizes different objects on the road, leading to safer navigation.
- Enhanced Situational Awareness: By understanding object attributes and relationships, the AI can make informed decisions, like predicting pedestrian movement or recognizing a red light.
- Adaptability to Different Scenarios: Diversely labeled data allows the AI to adapt to various weather conditions, lighting situations, and road types.
The Challenges and Future of Image Annotation
Despite its critical role, image annotation for self-driving cars presents some challenges:
- Data Volume: The sheer amount of data required to train AI models necessitates efficient and scalable annotation solutions.
- Data Ambiguity: Real-world scenarios can be complex. Annotators need clear guidelines to handle ambiguous situations, like partially occluded objects.
- Data Bias: Unbiased data is crucial. Mitigating bias in image selection and labeling is essential for safe and reliable self-driving car systems.
Fortunately, advancements in technology are addressing these challenges. Tools like automated annotation platforms and active learning can improve efficiency and accuracy. Additionally, initiatives to develop standardized labeling guidelines and promote diversity in datasets are also underway.
Conclusion
Image annotation remains the cornerstone of training self-driving cars. As autonomous vehicles inch closer to reality, the importance of high-quality labeled data will only increase. By continuously refining annotation techniques and fostering collaboration between data scientists, engineers, and annotators, we can ensure self-driving cars navigate the future safely and efficiently.