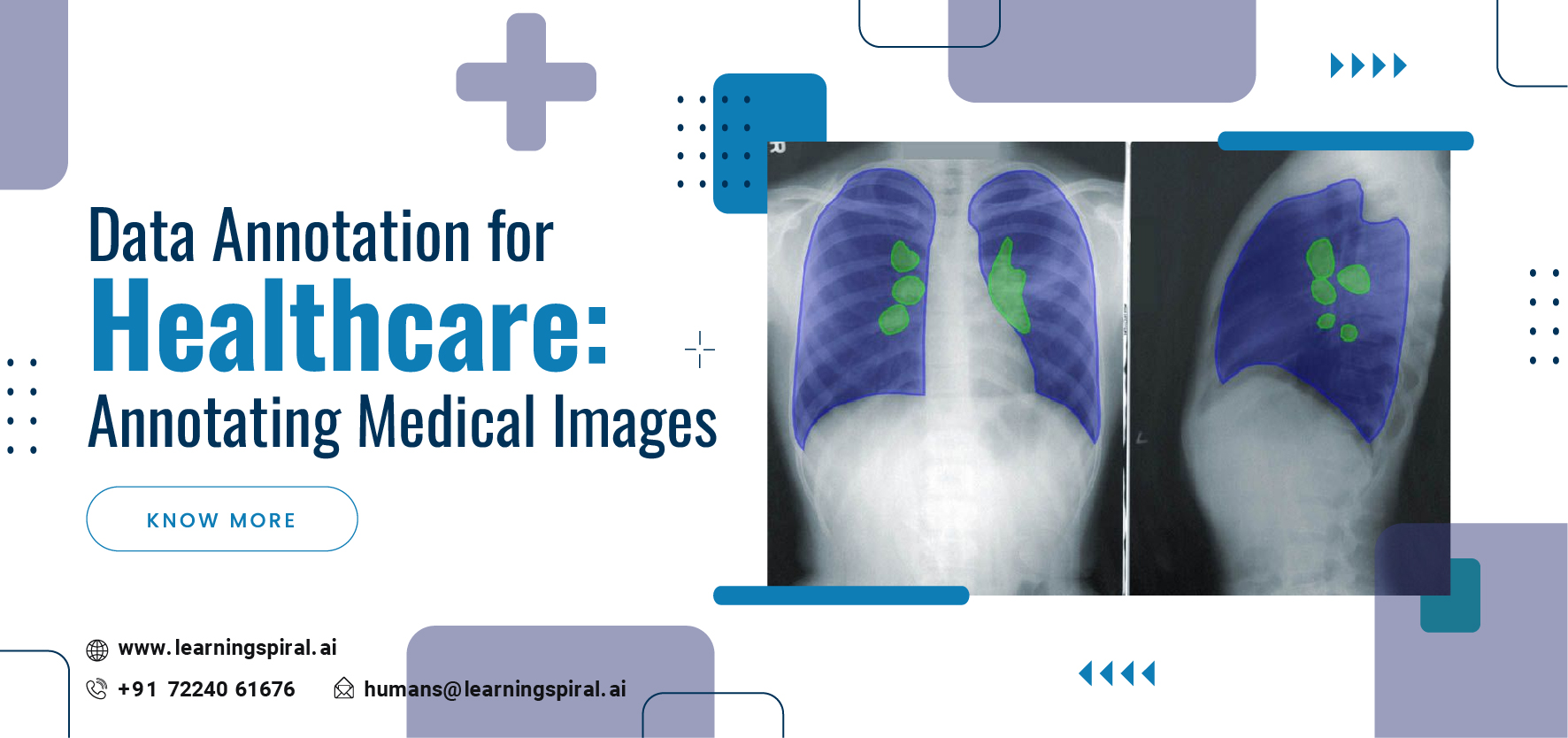
In today’s rapidly advancing healthcare sector, data plays a pivotal role in medical research, diagnosis, and treatment. Medical imaging, in particular, has seen remarkable advancements with the introduction of technologies such as MRI, CT scans, and ultrasound.
In healthcare, data annotation is used to enhance the quality of data and assist medical professionals in making more informed decisions. Here are some reasons why data annotation is critical in healthcare:
- Training Machine Learning Models: Machine learning models, aka AI, are increasingly used in healthcare for tasks like diagnosis and image analysis. Data annotation is crucial for training these models, as it provides labeled examples. These labels help the AI models and professionals learn to recognize and interpret medical images accurately.
- Improved Diagnosis: Accurate annotations on medical images help healthcare professionals in diagnosing diseases, conditions, and abnormalities. Annotations enable radiologists and other specialists to quickly identify and understand the features within the image, leading to faster and more precise diagnoses.
- Research and Development: Medical research relies heavily on data, and annotated datasets aid in the development of faster and well-detailed research files. This leads to the innovation of new treatments, drugs, and medical technologies. Researchers can use annotated medical images to investigate symptoms, study patient outcomes, and develop innovative solutions.
- Patient Monitoring: Annotated data can be used to monitor and track the progression of diseases and conditions over time. This enables healthcare providers to make necessary adjustments to treatment plans and improve patient care.
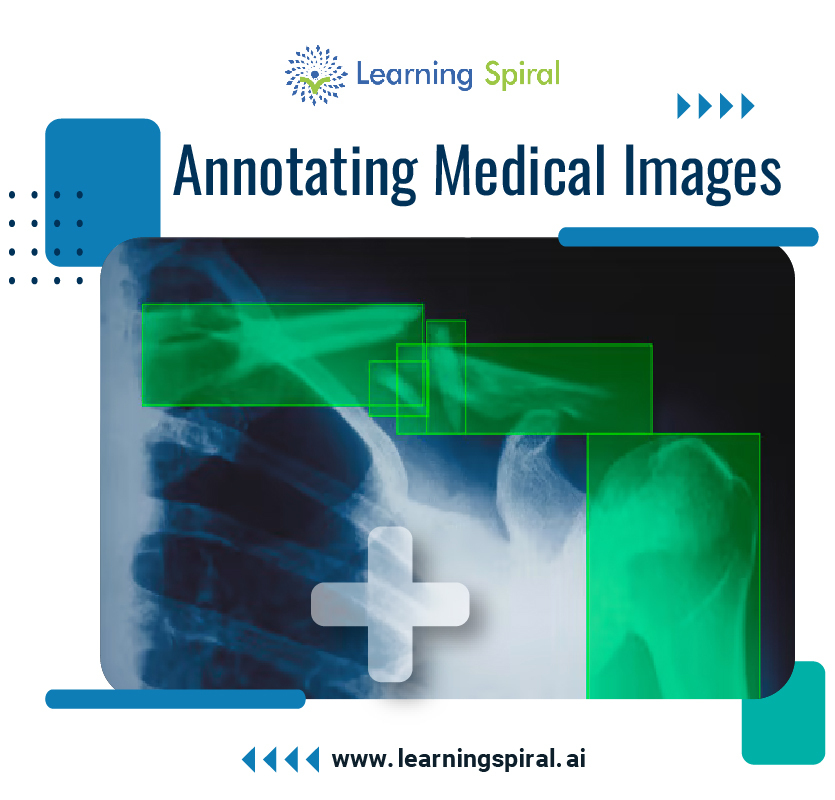
Annotating Medical Images
The annotation of medical images presents unique challenges due to the sensitive nature of the data and the need for high precision. Here are some of the challenges and solutions associated with annotating medical images:
- Data Privacy and Security: Medical images contain highly sensitive patient information. There have been examples of data being leaked and falling into the wrong hands. To address this challenge, vital data anonymization techniques need to be applied to protect patient privacy while still allowing for practical annotation.
- Variability in Annotations: Annotating medical images requires a high degree of accuracy and consistency. AI models are rumored to provide inaccurate data in large amount, leading to the creation of it being unreliable. Training annotation teams, using standardized guidelines, and implementing quality control measures can help ensure consistency in annotations.
- Large Datasets: The volume of medical images is vast, which can be overwhelming for manual annotation. Automation and semi-automation tools can help speed up the annotation process, making it more efficient.
- Interoperability: Ensuring that annotations are compatible with various healthcare systems and can be easily shared and integrated is crucial. Standardizing annotation formats and metadata can help enhance interoperability.
By observing the above challenges, data annotators could create better and more efficient outcomes to advance the medical imaging sector further. There is a huge scope for improvement with the collaboration of AI and the medical sector; all one needs is a push in the right direction.