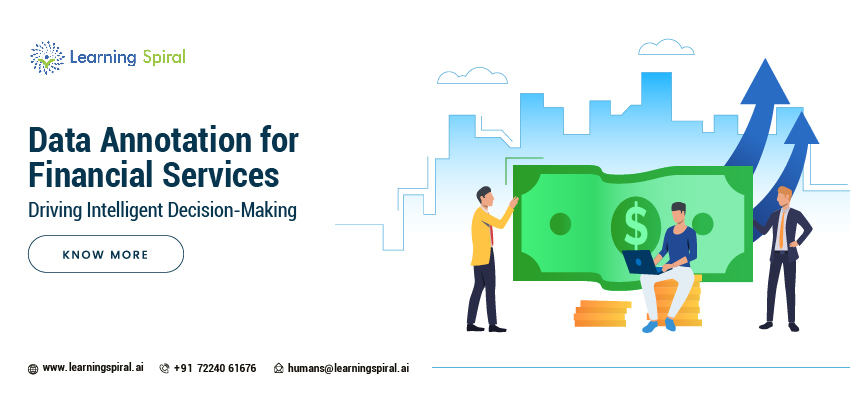
In today’s data-driven financial landscape, extracting meaningful insights from vast amounts of information is crucial for success. Data annotation, the process of labeling and classifying data, plays a critical role in empowering intelligent decision-making within financial services.
Importance of Data Annotation in Financial Services
Financial institutions generate a sea of data – transactions, documents, customer interactions, market trends. Data annotation transforms this raw data into a knowledge base for AI and machine learning (ML) models.
By labeling entities, categorizing documents, and tagging sentiment, the data becomes understandable and usable for algorithms. This empowers sophisticated analysis and decision-making beyond human capabilities.
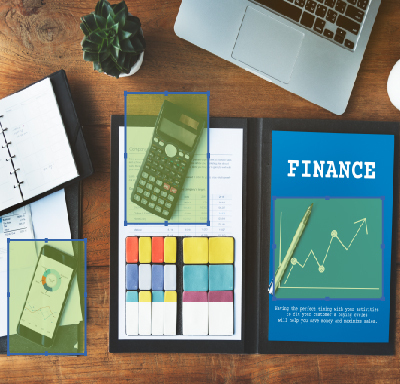
Data annotation fuels a multitude of applications within financial services:
- Fraud Detection: Machine learning models trained on annotated transaction data can identify anomalies and suspicious patterns in real-time, preventing fraudulent activities and saving millions.
- Risk Management: By analyzing labeled historical data, institutions can assess and predict creditworthiness, optimize lending decisions, and manage risk portfolios more effectively.
- Market Analysis: Annotating news articles, market reports, and social media data enables real-time sentiment analysis, uncovering market trends and predicting future volatility to inform investment strategies.
- Regulatory Compliance: Extracting key information from compliance documents through named entity recognition and other annotation techniques allows for automated compliance monitoring, reducing manual effort and ensuring adherence to regulations.
- Personalized Customer Service: Sentiment analysis within chat logs and customer interactions helps understand customer needs and preferences, enabling personalized financial advice and improved customer service experiences.
Difficulties in the Path
While data annotation unlocks its potential, ensuring quality and accuracy is paramount. Here are key considerations:
- Domain Expertise: Annotators need a deep understanding of financial concepts and specific regulatory nuances to ensure correct labeling and avoid biases.
- Standardization: Establishing consistent labeling processes and guidelines across teams prevents inconsistencies and enhances data reliability.
- Active Learning: Incorporating active learning techniques allows models to identify ambiguous data points and request annotations, improving labeling efficiency and model accuracy over time.
- Explainability: Implementing AI models that explain their reasoning fosters trust and understanding of decision-making processes, especially in critical areas like loan approvals.
Data annotation is not a static process but an ongoing journey. As financial data continues to grow exponentially, advanced AI-assisted annotation tools will play a significant role in streamlining the process, handling repetitive tasks, and ensuring quality. Collaboration between humans and AI in an active learning loop will lead to even more accurate and efficient data labeling.
Conclusion
In conclusion, data annotation is the hidden engine driving intelligent decision-making within financial services. By unlocking the power of data through accurate labeling, financial institutions can achieve greater efficiency, reduce risk, optimize operations, and deliver personalized services, paving the way for a more data-driven and successful future.