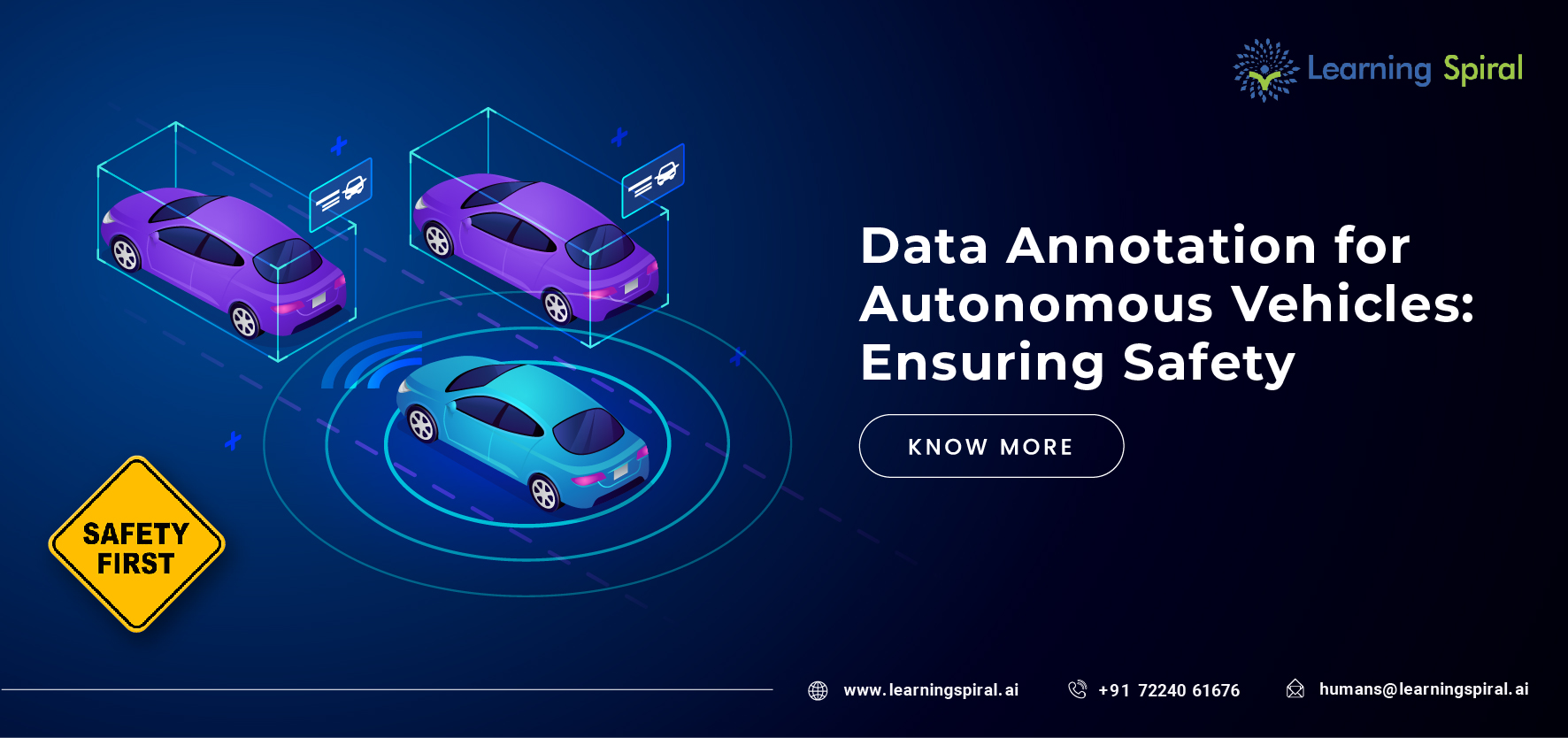
We are well aware of how the world is changing with the arrival and development of artificial intelligence. Sectors like education, healthcare, and even automobiles are now utilizing the new industry. Data annotation is thus playing a major role in making transportation safer and more convenient than ever.
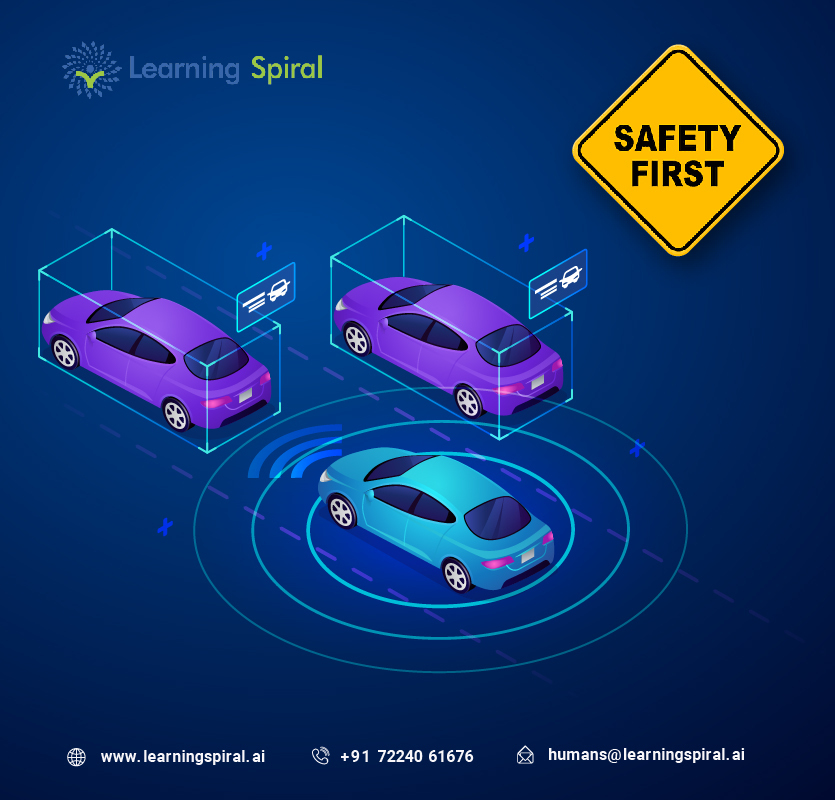
Accurate and well-annotated data is essential for training the vehicle’s machine learning algorithms, enabling it to make informed decisions and navigate safely. Here are some key aspects of data annotation for autonomous vehicles to ensure safety:
- Semantic segmentation: Annotating objects and their boundaries in images or sensor data, such as lanes, pedestrians, vehicles, and traffic signs. Accurate segmentation is vital for the vehicle to understand its surroundings.
- Object detection: Identifying and labeling objects in images or sensor data, such as cars, cyclists, pedestrians, and obstacles.
- Lane marking annotation: Annotating lane markings and road boundaries, helping the vehicle maintain its lane and navigate safely.
- Depth estimation: Providing depth information to help the vehicle understand the distance to objects in its path. This is critical for collision avoidance.
- Path planning: Annotating possible paths or trajectories for the vehicle to follow, taking into account traffic rules and safety considerations.
- Traffic sign recognition: Labeling traffic signs, signals, and their meanings to ensure the vehicle obeys traffic rules.
- Behavior prediction: Annotating the anticipated behavior of other road users (e.g., predicting if a pedestrian will cross the road), allowing the vehicle to make informed decisions.
- Map and localization data: Annotating high-definition maps and localization data, which can help the vehicle position itself accurately and navigate effectively.
- Weather and lighting conditions: Annotating data under various weather and lighting conditions (e.g., rain, snow, fog, nighttime) to train the vehicle to adapt to different environments.
- Anomaly detection: Annotating abnormal situations or potential hazards, such as road obstructions, accidents, or unexpected pedestrian movements.
- Diverse scenarios: Ensuring the dataset covers a wide range of scenarios, including urban, suburban, and highway driving, to prepare the autonomous vehicle for different environments.
- Sensor fusion: Annotating data from multiple sensors, including cameras, LiDAR, radar, and ultrasonics, to help the vehicle integrate information from various sources and make accurate decisions.
- Continual data updating: Regularly updating the annotated data to keep up with changing road conditions, construction zones, and new traffic patterns.
- Quality assurance: Implementing quality control measures to ensure accurate and consistent annotations, including double-checking by human annotators and using quality metrics.
- Machine learning feedback loop: Establishing a feedback loop to continuously improve the vehicle’s performance based on real-world data and user interactions.
- Ethical considerations: Ensuring the data annotation process considers ethical and privacy concerns, such as anonymizing sensitive information and respecting privacy regulations.
Data annotation is a critical but often overlooked aspect of autonomous vehicle development. Without the hard work of data annotators, self-driving cars would still be a distant dream.