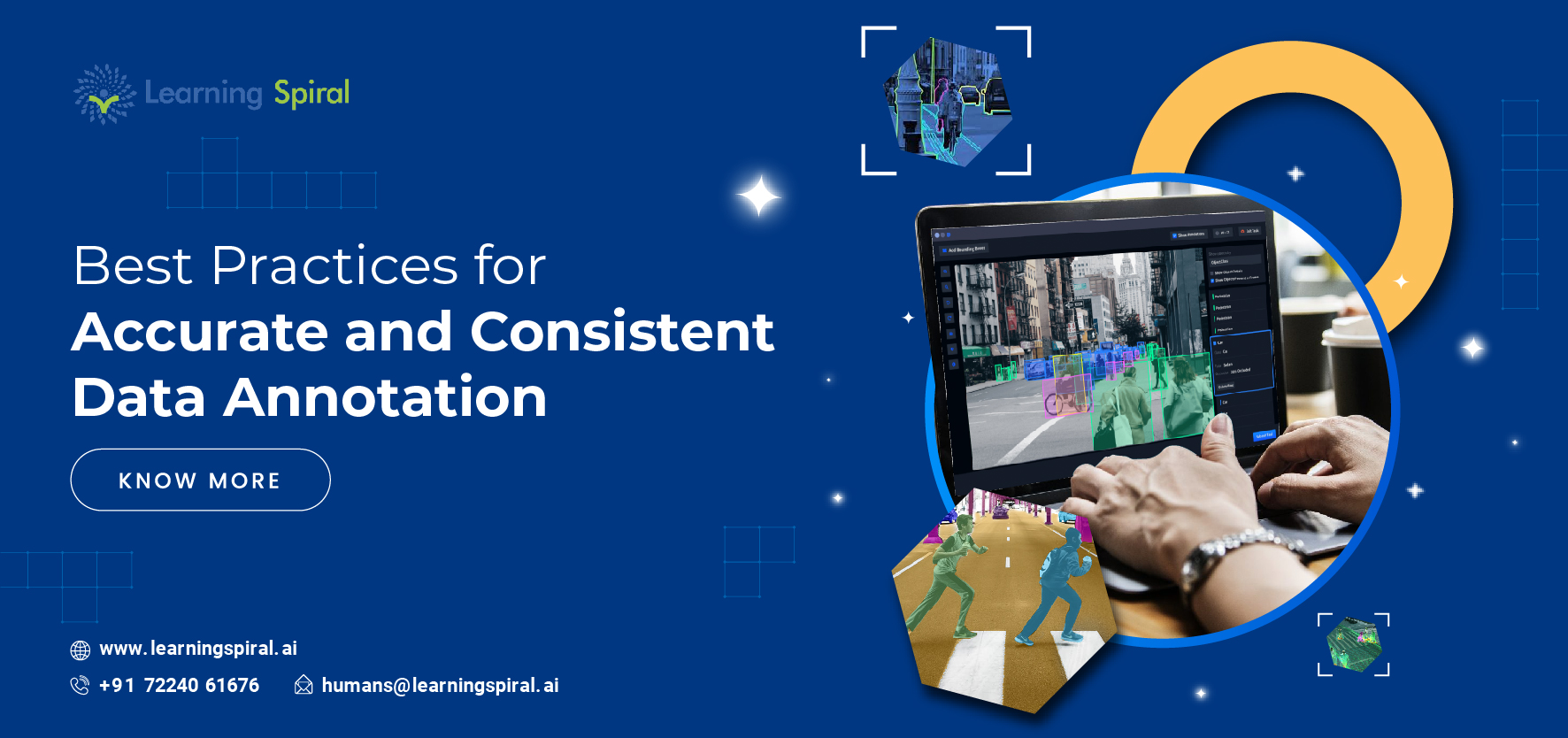
Data annotation is the process of labeling data with relevant information. It is done in several elements of image, text, audio and video data. It is a crucial step in the development of machine learning models, as the quality of the annotated data directly impacts the performance of the trained model.
Here are some best practices for accurate and consistent data annotation:
1. Defining Clear and Concise Annotation Guidelines
The guidelines provided for data annotation should be crystal clear with concise instructions. The following factors should be kept in mind while annotating data:
- A list of all possible labels and definitions
- Examples of how to apply each label
Keeping these factors in mind would allow even beginner annotators to avoid errors and guide the AI model in making the right decisions.
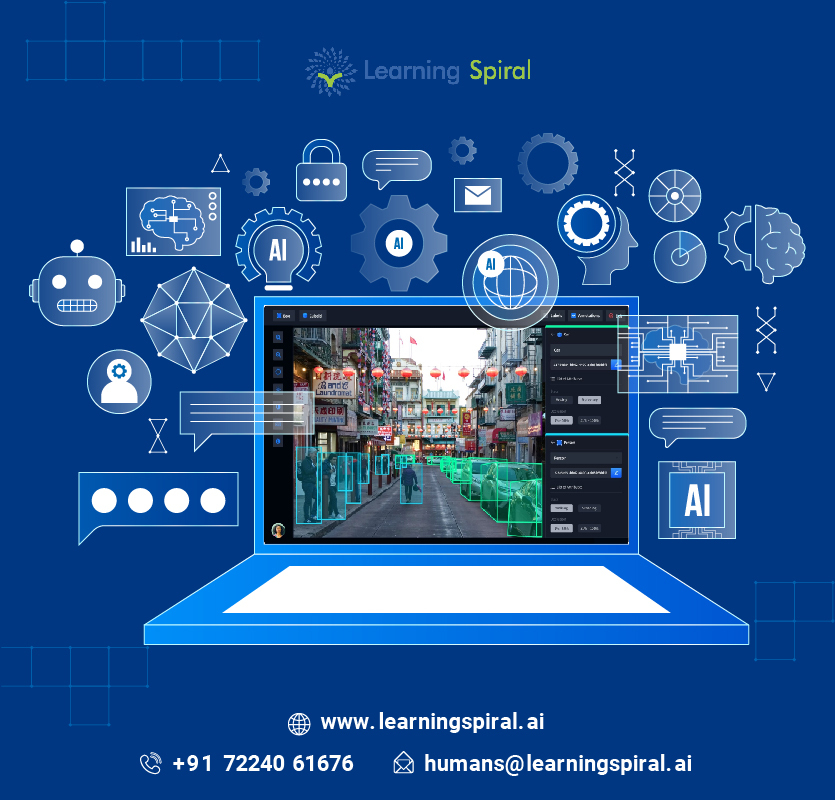
2. Select the Accurate Annotators
Two types of annotation are used to get the task done: one is manual, and the other is automated. Manual Annotators should have the necessary skills and knowledge to understand the data and apply the guidelines correctly. For example, if you are annotating medical images, you should select annotators with medical expertise.
Making sure that personal bias is not reflected in the annotated data is also important. As for automated annotators, the right guidelines and accurate resources are the key.
3. Use a Quality Assurance Process
Implementing a quality assurance process is important to ensure that the annotated data is accurate and consistent. For this, a hierarchy of annotators should be created, including an automated feature to check basic mistakes. This way, the data would be annotated by multiple individuals, reducing the possibility of errors to negligible.
4. Use the Right Annotation Tools
Various annotation tools are available in the market, each with its own strengths and weaknesses. Choose the tool that is best suited for your data type and annotation task. For this, businesses should hire the right support. Learning Spiral Pvt. Ltd. is one of the best data annotation companies in India. They have been providing guidance and services to big names in different sectors such as education, agriculture, health, etc.
5. Different Guidelines for Different Sectors
Every sector has different types of data, and those data need to be annotated slightly differently than others. For example, if you annotate images for a self-driving car model, you should create guidelines that include different objects, such as cars, pedestrians, and traffic signs. Notice to detail is what’s important while annotating data.
6. Bias Should be Avoided
If you are annotating text for a sentiment analysis model, you should create guidelines that define different sentiment labels, such as positive, negative, and neutral. Bias creation is one of the biggest problems AI tools create in the present time. To deal with these issues, the right direction is needed. The guidelines should also provide examples of how to label each sentiment, as well as edge cases, such as sarcastic or ambiguous text.
These are the practices one can follow to ensure accurate and consistent data annotation on a regular basis.