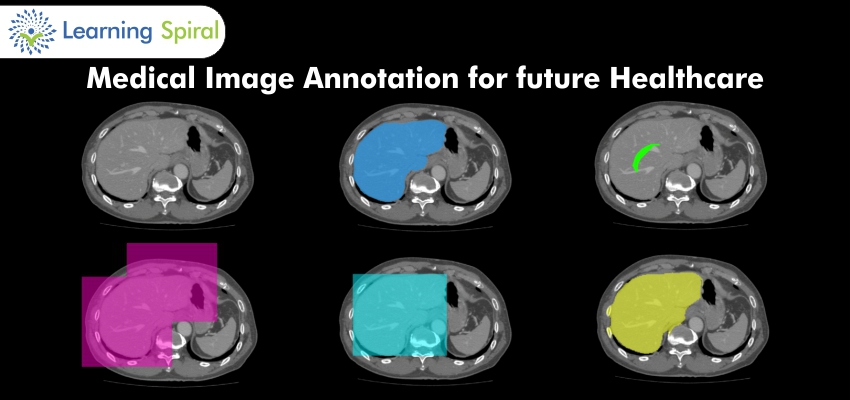
Image annotation is one of the most important tasks in computer vision. With numerous applications, computer vision essentially strives to give machine eyes – the ability to see and interpret the world. At times, machine learning projects seem to unlock futuristic technology we never thought would be possible. Talking of which, one of the major breakthroughs in which technology is piercing into is Healthcare. Training medical image datasets to feed machine learning algorithms.
Data annotation and data labeling tasks are Image Annotation provided by expert data annotation companies for many industries including the Medical sector. Medical Image annotation for future healthcare. Rather than other industries, the healthcare sector is totally different. It is on the high priority sector all of us expect more care and services without considering the cost. Most of the interpretations of medical data are being done by the medical expert after Data annotation.
How medical image annotation helps?
Medical Imaging Data using Semantic Segmentation
Medical image annotation service for machine learning healthcare data and big data healthcare training using semantic segmentation and polygon image annotation for organ segmentation and disease diagnosis.
AI-enabled devices can annotate pictures of medical imaging with the disorder in the body and on the basis of its image recognition capabilities, it will also automatically prepare the report after complete analysis and interpretation of results. Presently, such tasks are usually performed by humans and it could be a very difficult task for machines to predict accurate results right now. However, with the more improvements in AI-enabled diagnostic systems, medical imaging with machines will become more precise and accurate making it easier for medical professionals to make decisions and provide the best treatment to patients and so Medical data annotation is providing assistance to the medical industry
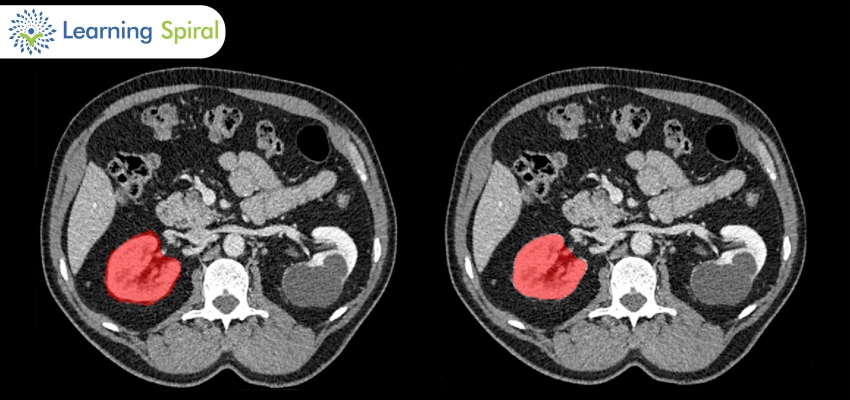
Medical images labeled or marked with certain techniques are called the image annotation that is done by data labeling company professionals to annotate the problem area in a medical image and outline the same with colored box, circle, or lines making it easily recognizable for computer vision.
Once the medical images are annotated, it is used at a large scale to train the machine learning or AI model. Using machine learning or deep learning algorithms is fed into the big data that helps to produce the right model that can itself analyze the medical images and predict what kind of disease is possible with the patient.
Thus, Medical image data annotation supports detecting and interpreting the changes in medical images It helps the radiologist to make better decisions. Hence it helps to save time and achieve the aim with minimal errors and discrepancies and so improves the accuracy.
Artificial-intelligence methods are moving into cancer research. Some recent case studies show the way new medical science getting better with the help of deep learning methods. Through this highly skilled technology doctors are able to track cancer cell movements and take actions on the preferred treatments. As cancer cells spread in a culture dish, Guillaume Jacquemet is watching. The cell movements hold clues to how drugs or gene variants might affect the spread of tumors in the body, and he is tracking the nucleus of each cell in a frame after frame of time-lapse microscopy films. But because he has generated about 500 films, each with 120 frames and 200–300 cells per frame, that analysis is challenging to say the least. “If I had to do the tracking manually, it would be impossible,” says Jacquemet, a cell biologist at Åbo Akademi University in Turku, Finland.
The American Heart Association published the results of a trial that shows stroke survivors are twice as likely to take anti-blood clot treatments when they are using an artificial intelligence (AI) platform, compared to those receiving more traditional treatment.
The AI platform, AiCure, uses software algorithms on smartphones to confirm patient identity, the medication, and if the medication was taken. Patients receive automated reminders and dosing instructions as well. Healthcare workers receive real-time data which allows for early detection of patients who are not taking their meds as scheduled.
“Many patients are unable to self-manage and are at increased risk of stroke and bleeding. The use of technology and artificial intelligence has the potential to significantly improve health outcomes and reduce costs in clinical care,” said Laura Shafner, study co-author and chief strategy officer at AiCure, in a press release.
AI has vast potential in the healthcare industry. It reduces the tasks that medical professionals must perform and that saves organizations money. Plus, there is plenty of data that healthcare generates, and AI systems can be trained to take advantage of this and provide useful information to healthcare providers.
There are more cases rising everyday where AI or ML is helping the patients who are suffering from either tumors or any other severe medical calamity.
Learning Spiral as a data annotation company hires professionals providing medical image annotation services to annotate medical images with the highest accuracy on AI-based models. and provide reliable medical data annotations services to help and support the healthcare industry in the most effective and efficient way.