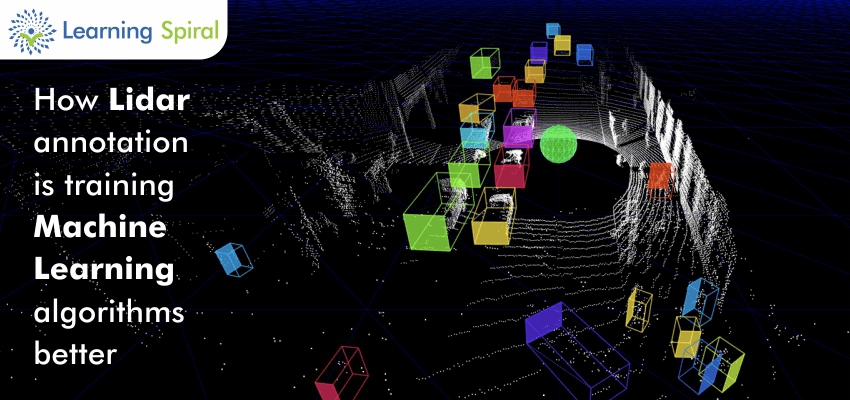
LIDAR Annotation and Labeling
LIDAR stands for LIght Detection and Ranging. It is a land surveying method that uses remote sensing technology emitting light that travels around the object and back to the receiver creating points every time it hits the object building a 3D map of the entire scene. We help annotate or label cars, pedestrians, bicyclists, trees, animals, traffic lights, billboards, garbage bin’s, etc in this map by drawing bounding boxes or cuboids precisely to train the machine learning algorithms to interpret the world. In short LIDAR Annotation: Identifies objects in a 3D point cloud and draws bounding cuboids around the specified objects, returning the positions and sizes of these boxes.
LiDAR annotation is similar to image labeling apart from the difference in practice for a simple reason: It is a 3D representation on a flat-screen. In addition, humans have to deal with a huge amount of points that are not contained by particular boundaries. So, even for professional humans, it is not easy to understand which point belongs to which object, and if you zoom into the point cloud image, this difficulty becomes clear. Even for LiDAR data, annotation is mostly done using the same ideas that guide the image labeling practices, such as bounding boxes or cuboids.
Lidar annotation is training ML algorithms better
LiDAR annotation technology is helping ML algorithms mainly by making semantic and instance segmentation of long sequences of LiDAR data highly efficient and accurate. Now it is possible to segment those long sequences in minimal time and with exceptional results, Lidar annotation INTEGRATED WITH MACHINE LEARNING is helping many ways to bring out efficient and accurate results. LiDAR has undergone major changes over the past years, and the most important thing is it has become increasingly very significant due to its fundamental role in autonomous vehicles to safely navigate our roads.
Learning Spiral provides high-quality data annotation services including professional image annotation services to companies so create AI space and train their models perfectly, perform better and get better results. Machine learning datasets are efficient enough to perform any high end automation. We provide efficiency to the machines. So, that is how the Robots work and communicate to the environment. We improve user experience by learning the machines from past experiences using historical data to solve problems and make decisions accurately.