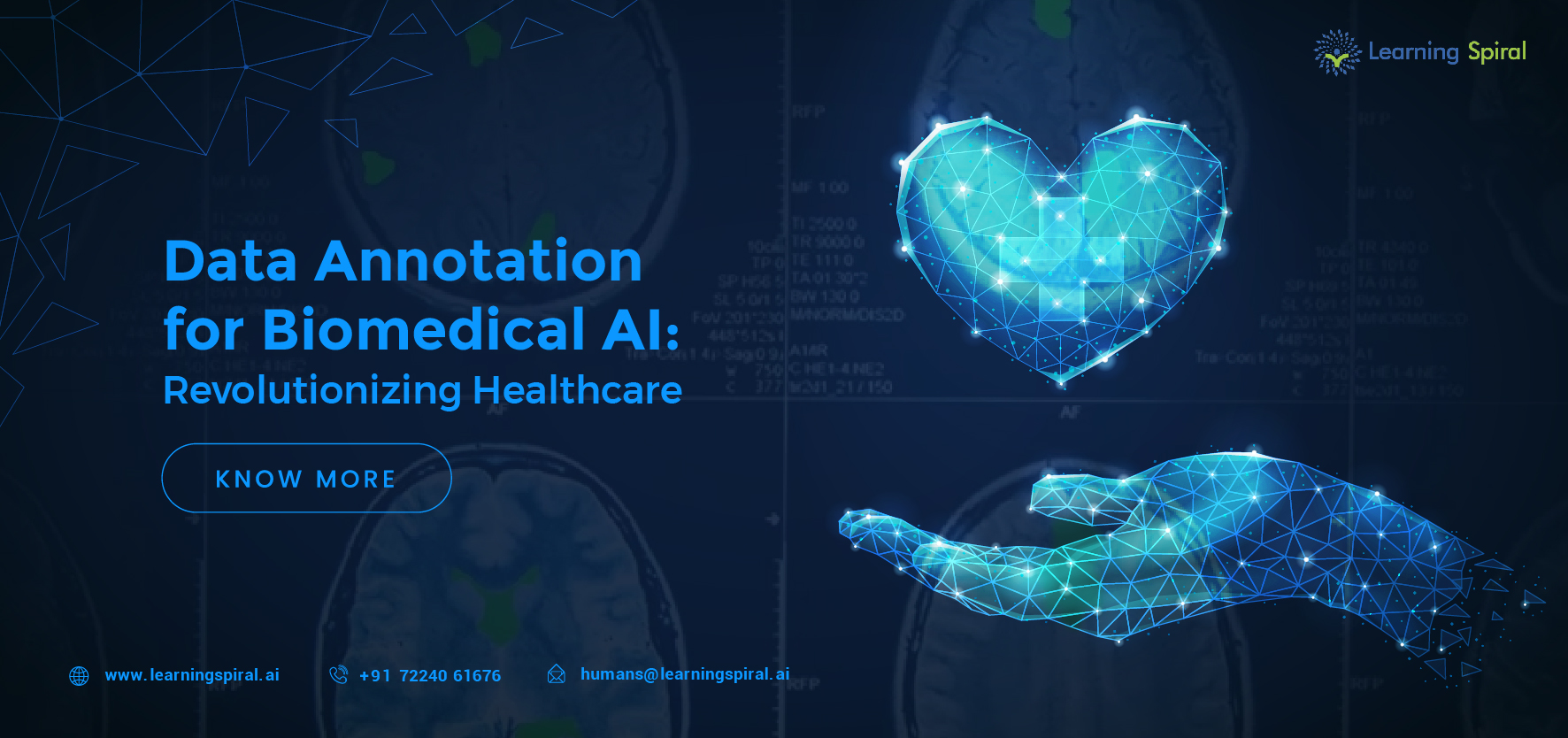
Artificial intelligence aka AI is rapidly transforming the healthcare landscape. From automating tasks to aiding in complex diagnoses, AI holds immense potential to improve patient care and outcomes. But continuing this development requires a crucial process: data annotation.
Data annotation acts as the bridge between raw medical data and powerful AI models. It involves meticulously labeling and structuring various forms of data, including medical images (X-rays, MRIs), text reports (patient histories, lab results), and even audio recordings. These annotations essentially provide context and meaning to the data, allowing AI models to learn and interpret the information accurately.
Here’s how data annotation is revolutionizing healthcare through AI:
1. Enhanced Diagnostic Accuracy:
One of the most promising applications lies in improved diagnostic accuracy. By training AI models on annotated medical images, we can empower them to detect abnormalities in scans with remarkable precision.
For instance, AI trained on annotated mammograms can highlight potential signs of breast cancer, assisting radiologists in early detection. Similarly, annotated pathology slides can be used to train AI for faster and more accurate cancer diagnosis.
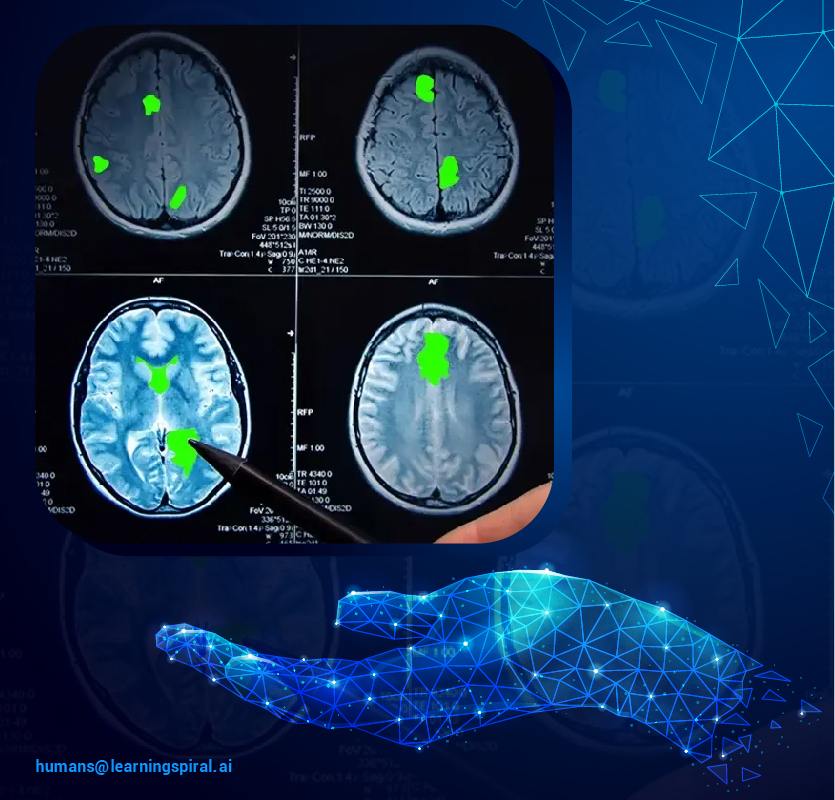
2. Personalized Medicine:
Data annotation plays a vital role in the development of personalized medicine. By analyzing vast amounts of annotated patient data, AI can identify patterns and variations that influence disease progression and treatment response.
This allows healthcare providers to tailor treatment plans to individual patients, maximizing effectiveness and minimizing side effects.
3. Early Disease Detection:
Early detection of diseases is critical for successful treatment. AI models trained on annotated data can analyze medical records and identify subtle signs of illness before symptoms even appear. This can lead to earlier intervention, potentially saving lives and improving patient prognosis. For example, AI trained on annotated retinal scans can detect signs of diabetic retinopathy, a leading cause of blindness.
4. Streamlined Workflow and Efficiency:
Data annotation empowers AI to automate various healthcare tasks, freeing up valuable time for medical professionals. AI can handle tasks like scheduling appointments, generating reports, and analyzing routine scans. This allows doctors and nurses to focus on more complex patient interactions and critical decision-making.
5. Accelerated Research and Development:
The healthcare field thrives on continuous research and development. Data annotation plays a key role here as well. By enabling AI to analyze large volumes of annotated medical data, researchers can gain deeper insights into diseases, identify potential drug targets, and accelerate the development of new treatments.
Challenges:
Despite its immense potential, data annotation for biomedical AI also faces challenges. Annotating medical data requires expertise and can be time-consuming. Ensuring the accuracy and consistency of annotations is crucial for reliable AI models. Additionally, ethical considerations regarding data privacy and security need to be addressed to safeguard sensitive patient information.
Conclusion
Data annotation is a critical but often unseen force driving the AI revolution in healthcare. As we move forward, advancements in automation and crowdsourcing can streamline the annotation process. Moreover, fostering collaboration between data scientists, medical professionals, and AI developers is essential to ensure the ethical and effective use of annotated data for a healthier future.
By overcoming these challenges and harnessing the power of data annotation, we can truly unlock the potential of AI to revolutionize healthcare and improve patient outcomes on a global scale.