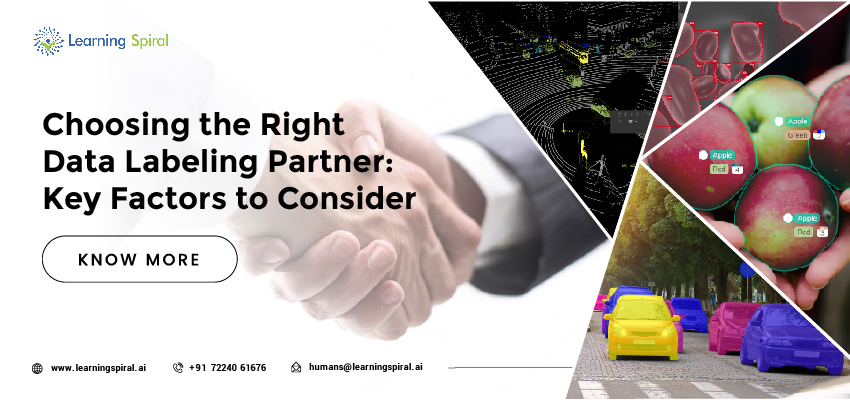
In the age of AI, data labeling plays a vital role in training machine learning models. High-quality labeled data is the fuel that powers accurate and efficient AI systems.
However, the data labeling process itself can be complex and requires expertise in specific domains. This is where partnering with a reliable data labeling company becomes crucial.
Selecting the right partner can significantly impact the success of your AI project. Here are some key factors to consider when choosing a data labeling company:
1. Expertise and Experience:
When it comes to expertise, these two parameters are important to check:
- Domain Knowledge:
Does the company have experience labeling data specific to your industry or application? For example, medical imaging data labeling requires expertise in healthcare terminology, while labeling for autonomous vehicles requires understanding traffic rules and object recognition.
- Project Complexity:
Can the company handle the complexity of your data labeling needs? Simple tasks like image bounding boxes might be suitable for general providers, while complex tasks like semantic segmentation require specialized experience.
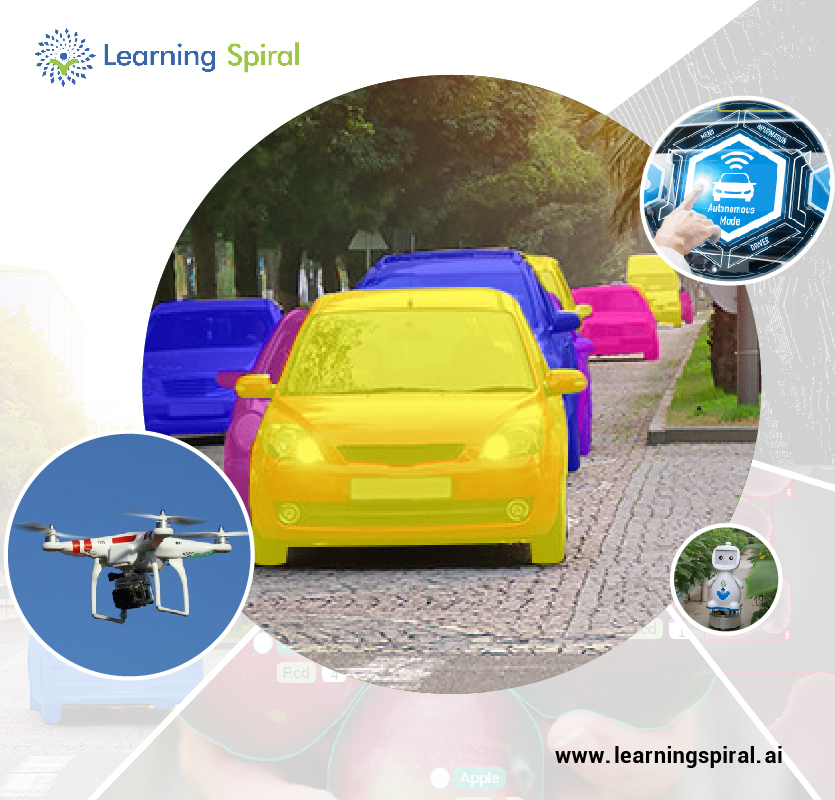
2. Data Quality and Security:
Make sure to check these factors when it comes to security and quality:
- Quality Control Measures:
How does the company ensure the accuracy and consistency of labeled data? Look for providers with robust quality control processes, including double-annotation, peer review, and inter-rater reliability checks.
- Data Security:
What measures are in place to protect your sensitive data? Ensure the company adheres to industry best practices for data security, including encryption, access control, and regular security audits.
3. Cost and Pricing Models:
Pricing is essential while choosing the right company:
- Pricing Transparency:
Does the company offer transparent pricing models with clear cost structures? Beware of hidden fees or lack of clarity about project costs.
- Scalability and Flexibility:
Can the company scale their resources to meet your growing data labeling needs? Look for providers with a scalable workforce and flexible pricing models that accommodate project fluctuations.
4. Technology and Infrastructure:
Analyzing the features and tools of the company is extremely important:
- Labeling Tools and Platforms:
What data labeling tools and platforms does the company use? Modern platforms offer features like annotation tools, data management functionalities, and workflow management capabilities.
- Scalable Infrastructure:
Does the company have the infrastructure to handle large and complex datasets? Adequate infrastructure ensures efficient data processing and timely project completion.
5. Communication and Support:
- Communication Channels:
How will the company communicate with you throughout the project? Clear and regular communication is essential for project management and addressing any issues that might arise.
- Support and Training:
Does the company offer support and training to ensure a smooth collaboration? Look for providers that offer project management expertise and training for your team on using their platform.
- Location and Time Zone:
Consider the location and time zone of the data labeling team. A well-aligned time zone can facilitate communication and ensure efficient project management.
By carefully considering these factors, you can choose a data labeling partner that best suits your project needs. It’s also advisable to request proposals from several vendors, compare their offerings, and conduct reference checks to gain insights into their past performance.
Conclusion
Choosing the right data labeling partner is an investment in the success of your AI project. Learning Spiral Pvt. Ltd. excel in covering most (if not all) of these factors in its services to ensure secure, efficient and smoothest labeling option.
By prioritizing expertise, data quality, cost-effectiveness, technology, and communication, you can ensure a smooth and efficient data labeling process, ultimately leading to the development of robust and accurate AI models.