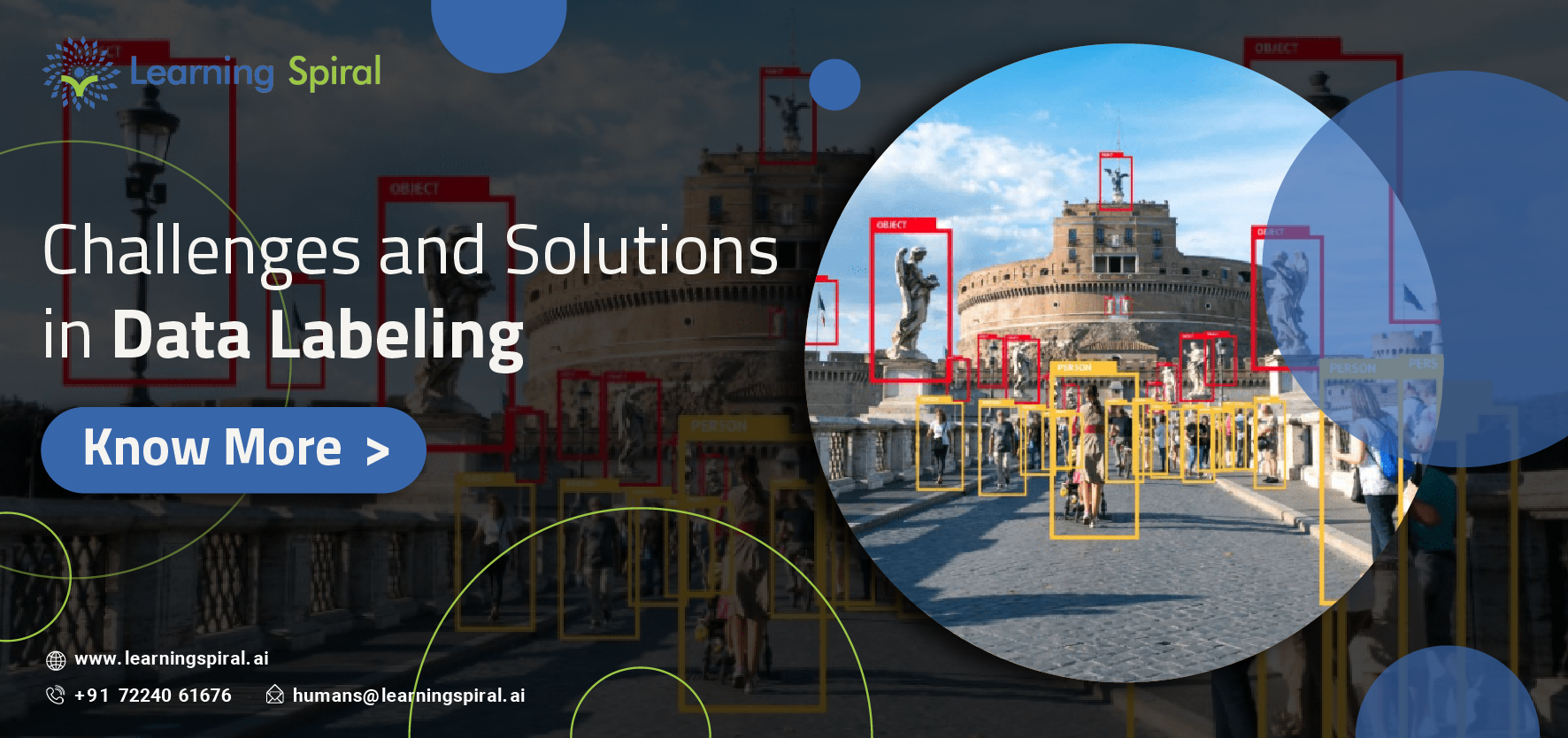
An important tool in the AI world, data labeling is the process of assigning labels to data points, such as text, images, or audio. These data labels are then used for machine learning and artificial intelligence (AI) applications. In simple words, the labels allow the machine to identify various points or elements in the data and perform its task wisely. It is a critical step in the development of AI models, but it can also be a challenging and time-consuming process.
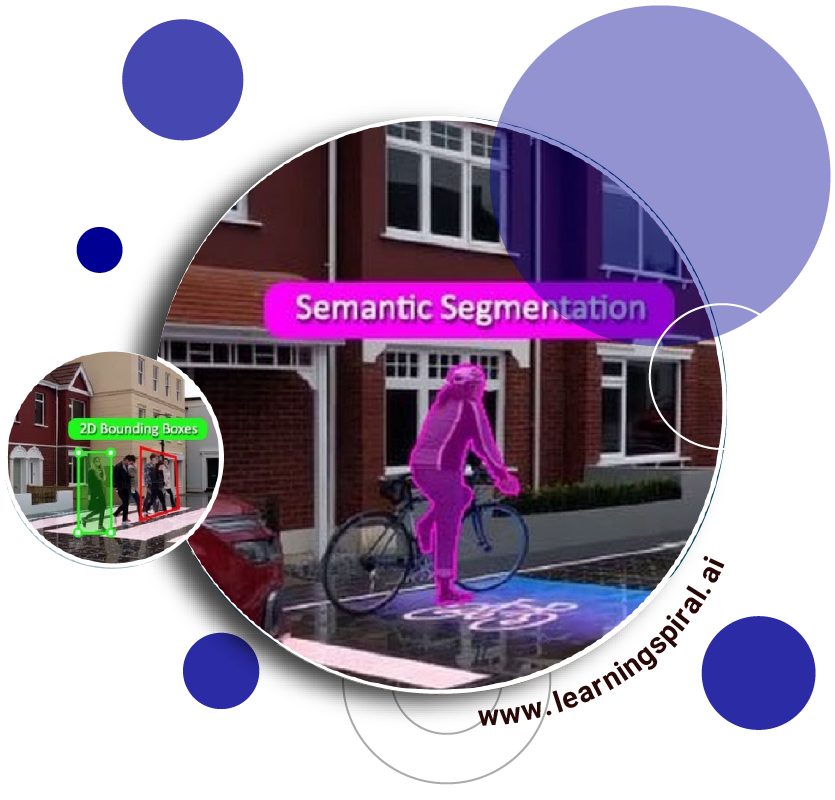
In this article, we will check out some challenges one might face while going through data labeling and what their solutions are.
Challenges in Data Labeling
There are a number of challenges associated with data labeling, including:
- Data volume: The one big issue with data labeling is that training a large language model often requires millions or even billions of labeled data points. Thus, the procedure can be very tedious and complicated with baggage of data.
- Data complexity: With a load of data labels come complexities of varying kinds. For example, images may contain multiple objects or people, and text may be ambiguous or contain slang terms.
- Data quality: This is the one issue every content platform suffers from, so how can AI tools avoid this? The data can be incorrect or incomplete. If the data is not labeled accurately, it can lead to errors in AI models.
Solutions to the Challenges in Data Labeling
There are a number of solutions that can help to address the challenges of data labeling. These include:
- Automated Data Labeling: Solving the crisis of large time-taking and tremendous costs, automated data labeling tools often come in handy. One such platform is the Learning Spiral. Get your AI models’ labeling done for complex to large datasets with ease.
- Human-in-the-Loop Labeling: When human makes mistakes, AI comes in handy. This theory can be reversed as well. Human-in-the-loop labeling is a hybrid approach that combines automated data labeling with human review. This approach can help to improve the accuracy of labeled data so errors can be avoided.
- Start with a small, well-defined dataset: It is often helpful to start with a small, well-defined dataset when labeling data. This will help you to identify and address any challenges early on.
The challenges of data labeling are significant, but there are a number of solutions that can help to address them. By using a combination of automated and human-in-the-loop labeling methods, organizations can improve the accuracy and efficiency of data labeling, and ultimately build better AI models.
There is no single best way to label data. By using a variety of methods, such as automated labeling, human-in-the-loop labeling, and crowdsourcing, one can realise which method suits them best. By following these tips, you can help to overcome the challenges of data labeling and build better AI models.